By Vinesh Jha, CEO & founder of ExtractAlpha
.
Are you interested in developing a new or fine tuning your existing systematic investing process to produce more alpha? Then, this series was written for you … here’s the first of three posts about systematic investing, and data science best practices. You’ll learn why a systematic approach is imperative, and why failing to be systematic can be dangerous. Subsequent posts will focus on best practices in systematic investing and some common pitfalls.
As a systematic portfolio manager and researcher – a quant or data scientist – it’s easy to forget that being systematic doesn’t come easily to everyone. Many experienced portfolio managers do not come from a quantitative background, and although they may have a long tenure in financial markets, they may not have thought about investing problems in the same way as a quant like myself has.
This is not to discount the advantages of using the intuition of the human brain for investing. On the contrary, the best quant investing relies heavily on our brains’ intuition about economics, human behavior, statistics, and other market participants, and is often inspired by our fundamental counterparts. But the human brain is prone to building belief systems around anecdotes, and while anecdotes may have been useful in a world without rich data, they can be dangerous in a world in which we can do much better, and in which some of our competitors are sure to be doing better.
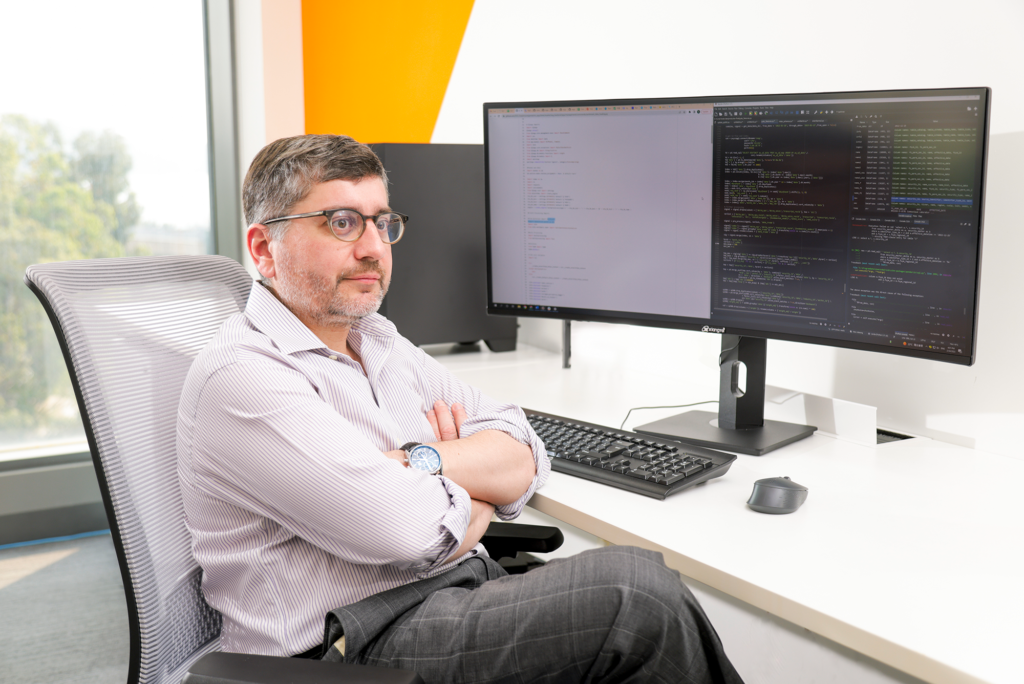
Vinesh Jha, 2023. As a quant, I am devoted to identifying alternative datasets, making them easily digestible, and delivering them as raw data and trading signals.
Anecdotal evidence isn’t evidence
Anecdotes fail us because they provide a compelling and safe story, but are not borne out by proper data analysis. For example we may observe something consistently (or so we think), but we may attribute it to the wrong underlying cause. A drop in reported nationwide unemployment numbers may on its surface seem to be unequivocally good, but the headline numbers obscure underemployment as well as the fact that many unemployed workers have simply stopped looking for a job and therefore have stopped applying for unemployment benefits – which unemployment stats are based on.
We may also see what we want to see, in what behavioral economists refer to as Confirmation Bias: we think IPOs outperform because we hear about the big famous ones that “pop” – but on average, companies going public actually underperform! It turns out that this is because managers cash out when they perceive their stocks to be overvalued by the market. But you’d never realize this reading the news.
The investment world is increasingly data-driven. Even fundamental managers are increasingly embracing quantitative techniques and alternative data sources in their decision making process. This is potentially great – data science gives us excellent tools for portfolio and risk management – but also potentially quite dangerous if those tools are used incorrectly.
More butter is better
By being systematic we can address common biases and design proper research so we can learn about the markets in a more rigorous way. But we have to be cognizant of certain common pitfalls in data analytics. It’s easy to call yourself a data scientist these days, and much harder to really act scientifically when it comes to noisy market data.
As a quick example, David Leinweber famously wrote about his finding that butter production in Bangladesh was the best explanatory variable for S&P 500 returns… at least during some arbitrary time period. It should be clear why his finding was (intentionally) bunk, but overfitting of this kind is just one of several types of common research errors. In the post, we’ll address overfitting and some other common issues, and then talk about some of the best practices we use at ExtractAlpha to enable researchers to overcome common biases and pitfalls.
To read Part II, click here. To be notified of new content, please subscribe (see below) or follow us on LinkedIn!