Data analysis stands as a cornerstone in our increasingly data-oriented world, offering a beacon of insight that drives informed decision-making across various fields.
Whether it’s boosting business efficiency, enhancing financial strategies, or advancing scientific research, the ability to parse through vast datasets and extract meaningful information is invaluable. This article demystifies the process by delineating the seven essential steps of data analysis.
From the initial stage of defining clear objectives to the final steps of interpreting results and communicating insights, we provide a structured roadmap that equips readers with the necessary tools to navigate the complex landscape of data analysis effectively. By following this systematic approach, you can unlock the full potential of your data, transforming raw numbers into actionable strategies that propel informed decisions.
1. Define Objectives and Goals
The first and perhaps most crucial step in the data analysis process is defining your objectives and goals. This foundational step sets the stage for all subsequent actions and decisions, guiding the scope and direction of your analysis. Without a clear understanding of what you aim to achieve, the data exploration can become unfocused and inefficient, leading to ambiguous or non-actionable insights.
What are Objectives and Goals?
Objectives articulate the purpose of your data analysis. They are precise, measurable statements that define what you intend to accomplish through your analysis. Goals, while similar, are broader and provide a general direction rather than specific endpoints.
Key Considerations for Defining Objectives and Goals:
- Specificity: Objectives should be as specific as possible. Instead of a broad objective like “increase business efficiency,” specify what aspects of efficiency you’re focusing on—perhaps “reduce delivery times by 15% within the next quarter.”
- Relevance: Ensure that the objectives align with broader business or research goals. The insights gleaned should have practical implications that resonate with overarching strategies.
- Feasibility: Consider the availability of data and resources. Setting objectives that are beyond the scope of your current data infrastructure or analytical capabilities can lead to frustrations and wasted efforts.
- Measurability: Define how you will measure success. What metrics will indicate whether you’ve achieved your objectives? This might include increases in customer satisfaction scores, reductions in costs, or improvements in operational speed.
- Timeline: Establish a realistic timeline for achieving your objectives. This helps in maintaining momentum and setting expectations for stakeholders.
Practical Steps to Define Objectives and Goals:
- Brainstorming Session: Gather key stakeholders and conduct brainstorming sessions to capture diverse perspectives and needs.
- Alignment with Vision: Ensure that the data analysis objectives are well-aligned with the broader organizational or project vision.
- Literature Review: Conduct a review of industry benchmarks, previous research, or case studies to inform and refine your objectives.
- Draft and Refine: Draft your objectives and then refine them based on feedback from stakeholders and alignment with data capabilities.
- Documentation: Clearly document the finalized objectives and goals to ensure that everyone involved in the analysis has a common understanding.
By rigorously defining the objectives and goals of your data analysis, you lay a strong foundation for all subsequent steps, ensuring that your efforts are well-directed and aligned with specific, measurable outcomes. This clarity not only optimizes the analysis process but also enhances the applicability and impact of the insights generated.
2. Data Collection and Preparation
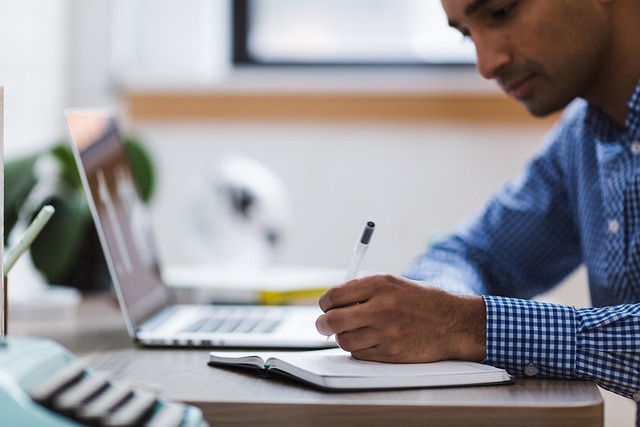
The second step in the data analysis process involves collecting and preparing data, which serves as the backbone for all analytical tasks that follow. This stage ensures that you have relevant, clean, and organized data to work with, which can significantly influence the accuracy and reliability of your analysis outcomes.
Gather Relevant Data
Identifying Data Needs: Firstly, determine the type of data required to meet your analysis objectives. This may include demographic information, transactional data, sensor outputs, among others.
Sources of Data:
- Internal Sources: These include databases, CRM systems, and other internal reports that your organization maintains.
- External Sources: Public data sets, purchased data, online scraping, and APIs from third-party providers.
Data Collection Methods:
- Automated Data Collection: Utilizing scripts or tools to collect data periodically from databases or APIs.
- Manual Data Collection: Involves surveys, interviews, or manual entry where automated methods are not feasible.
Ensuring Data Quality: Data must be relevant, complete, and accurate. Verify the data against known sources for accuracy and check for completeness.
Clean and Format Data
Data Cleaning:
- Handling Missing Values: Decide whether to fill in missing values using interpolation or imputation, or to remove rows or columns with missing data.
- Removing Duplicates: Identify and remove any duplicate records to prevent skewed analysis results.
- Dealing with Outliers: Identify outliers and decide whether they represent special cases or data errors. Handling outliers might involve modifications or removal.
Data Formatting:
- Standardizing Data Formats: Ensure that all data types are consistent (e.g., dates in the same format, categorical variables handled uniformly).
- Normalization: Scale numeric data to a consistent range if required, especially relevant in machine learning models.
Creating a Data Preparation Pipeline: To streamline the process, develop a data preparation pipeline that automates as many of these tasks as possible. This pipeline can include scripts for cleaning, transformations, and formatting, which can be reused for similar future analyses.
Validation and Documentation: After cleaning and formatting, validate the dataset to ensure it meets the quality standards set for the analysis. Document the steps taken during the data collection and preparation phase for transparency and reproducibility.
3. Data Exploration
After assembling and refining your dataset, the next vital step in the data analysis process is data exploration. This phase helps analysts understand the underlying structure of the data, identify patterns, anomalies, or inconsistencies, and form hypotheses for deeper analysis. Effective data exploration can reveal insights that guide the entire analytical approach, influencing how data will be further processed and analyzed.
Understanding Your Data
- Descriptive Statistics: Begin with basic descriptive statistics such as mean, median, mode, range, and standard deviation. These metrics provide insights into the central tendency and variability of the data.
- Distribution Examination: Analyze the distribution of your data. This includes identifying the shape of the distribution (normal, skewed, bimodal) and detecting any potential anomalies that could affect further analysis.
- Correlation Check: Evaluate the relationships between variables. Correlation coefficients can highlight direct or inverse relationships, informing potential causality or influence between variables.
Visual Data Analysis
- Data Visualization Tools: Utilize graphical representations to better understand and present the data. Common tools and techniques include:
- Histograms and Box Plots: Useful for visualizing data distributions and spotting outliers.
- Scatter Plots: Ideal for observing relationships and trends between two variables.
- Heatmaps: Effective for visualizing correlation matrices or two-way table data.
- Time Series Plots: Essential for data with a temporal dimension, to identify trends, cycles, or irregular patterns.
Exploratory Data Analysis Techniques
- Segmentation and Stratification: Divide the data into subsets based on certain criteria (e.g., demographic groups in marketing data) to explore specific behaviors within and across these segments.
- Principal Component Analysis (PCA): A technique used to reduce the dimensionality of the data set, increasing interpretability while minimizing information loss.
- Clustering: Apply clustering algorithms to group similar data points together, which can reveal hidden patterns and categorizations within the data.
Forming Initial Hypotheses
Based on the observations and findings from the exploration phase, start forming hypotheses about the data. These hypotheses might relate to:
- Causes of observed phenomena.
- Predictions about future data behavior.
- Relationships between variables that may be tested in more detail during the modeling stage.
Identifying Data Quality Issues
- Consistency Checks: Look for any inconsistencies or illogical values within the data that might indicate quality issues.
- Completeness Assessment: Evaluate if there are significant gaps in the data that could impact the analysis outcomes.
Preparing for Next Steps
Data exploration often uncovers additional data needs or preprocessing steps. You may need to return to earlier stages to refine your dataset based on insights gained during exploration. Documentation during this phase is crucial, as it supports the traceability of insights and facilitates collaborative analysis efforts.
4. Data Preprocessing
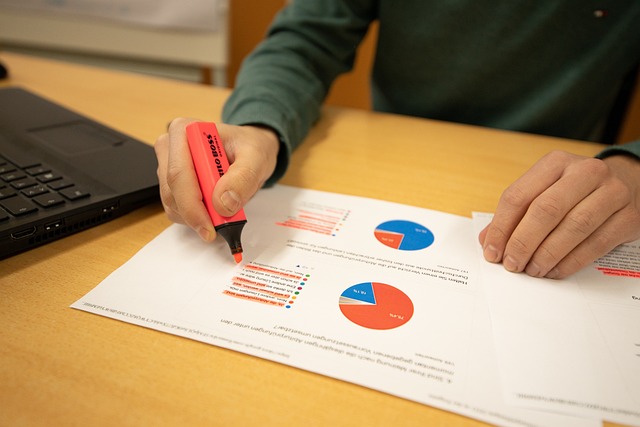
Data preprocessing is a critical step in the data analysis process that prepares the raw dataset for modeling and analysis. This phase involves transforming and fine-tuning data into a format that enhances the effectiveness of machine learning algorithms or statistical models. Proper data preprocessing not only improves the quality of data but also significantly boosts the accuracy and efficiency of the subsequent analytical results.
Feature Engineering
Feature engineering is the process of using domain knowledge to create new features from raw data that help algorithms to better understand the underlying patterns in the data.
- Creating Interaction Features: Combine two or more variables to create a new feature that captures their combined effects on the predictive model.
- Polynomial Features: Generate new features by considering non-linear relationships of the existing data.
- Binning/Bucketing: Convert continuous variables into categorical counterparts by dividing the data into bins or intervals, which can help in handling outliers and non-linear relationships.
- Encoding Categorical Variables: Transform categorical variables into numerical format through one-hot encoding, label encoding, or similar techniques, making them interpretable by machine learning models.
- Dimensionality Reduction: Techniques like PCA (Principal Component Analysis) are used to reduce the number of variables under consideration, by extracting the principal components that capture the most variance in the data.
Scaling and Normalization
Many algorithms perform better when numerical input variables are scaled or normalized. This ensures that the model treats all features equally, especially when they are measured in different scales.
- Standardization (Z-score Normalization): Subtract the mean and divide by the standard deviation for each data point. This centers the data around zero and scales it to unit variance.
- Min-Max Scaling: Rescale the feature to a fixed range, usually 0 to 1, by subtracting the minimum value and dividing by the maximum minus the minimum.
- Normalization: Scale individual data samples to have unit norm. This type of scaling is often used when using dot products or other algorithms that measure distances between data points.
Handling Missing Values
- Imputation: Replace missing values with a statistical value derived from the non-missing values, such as the mean, median, or mode. For more sophisticated approaches, you can use regression, or methods like k-nearest neighbors, to predict and fill missing values.
- Removal: In cases where the percentage of missing data is high, or imputation would introduce significant bias, consider removing the affected rows or columns entirely.
Missing data can mislead or distort the performance of models if not addressed properly.
Data Transformation
Transforming data includes a variety of techniques aimed at making the data more suitable for modeling.
- Log Transformation: Useful for dealing with skewed data, helping to stabilize variance and normalize data.
- Power Transforms: Generalize the log transformation, and include options like square root or reciprocal that can help in normalizing data distributions.
Random Sampling
When dealing with particularly large datasets, it might be practical to sample the data randomly to reduce computational costs during the exploratory and modeling phases, provided that the sample represents the larger dataset accurately.
5. Data Modeling
Data modeling is a pivotal step in the data analysis process where the prepared data is used to build models that can predict, classify, or provide insights into the data based on the analysis goals defined earlier. This phase involves selecting appropriate modeling techniques, training models, and evaluating their performance to ensure they meet the specified objectives.
Selection of Modeling Techniques
- Understanding the Problem: The choice of modeling technique largely depends on the nature of the problem (e.g., classification, regression, clustering).
- Algorithm Selection: Choose algorithms that best suit the problem’s requirements and the data characteristics. Common choices include linear regression for continuous outcomes, logistic regression for binary outcomes, decision trees, support vector machines, and neural networks for more complex data structures.
- Ensemble Methods: Consider using ensemble methods like Random Forests or Gradient Boosting Machines (GBM) that combine multiple models to improve prediction accuracy and robustness.
Splitting the Dataset
- Training and Test Sets: Divide the data into training and test sets to evaluate the effectiveness of the models. Typically, the data is split into 70-80% for training and 20-30% for testing.
- Cross-Validation: Implement cross-validation techniques, especially k-fold cross-validation, to ensure that the model’s performance is consistent across different subsets of the data.
Model Training
- Parameter Tuning: Optimize model parameters, often referred to as hyperparameter tuning, to enhance model performance. Techniques like grid search or random search can be helpful.
- Feature Importance: Evaluate the importance of different features in the model, which can provide insights and may lead to further feature engineering or selection.
Model Evaluation
- Performance Metrics: Choose appropriate metrics to assess model performance based on the type of analysis. For regression models, metrics might include Mean Absolute Error (MAE) or Root Mean Squared Error (RMSE). For classification tasks, metrics like accuracy, precision, recall, and the F1-score are commonly used.
- Validation: Assess the model performance using the test set or through cross-validation to ensure that the model generalizes well to new data.
- Diagnostic Measures: Analyze residuals and check for patterns that might suggest model inadequacies or opportunities for improvement.
Model Refinement
- Iterative Process: Model building is an iterative process. Based on the evaluation, return to previous steps to reconfigure the model, adjust features, or even revisit the data preprocessing phase.
- Simplicity vs. Complexity: Balance the complexity of the model with the need for simplicity. More complex models might perform better on training data but can overfit and perform poorly on unseen data.
Deployment
- Integration: Once validated and refined, integrate the model into the data analysis pipeline for deployment in real-world scenarios or decision-making processes.
- Monitoring: Continuously monitor the model’s performance over time to catch any degradation or shifts in data, which might necessitate retraining or adjustments.
6. Interpretation of Results
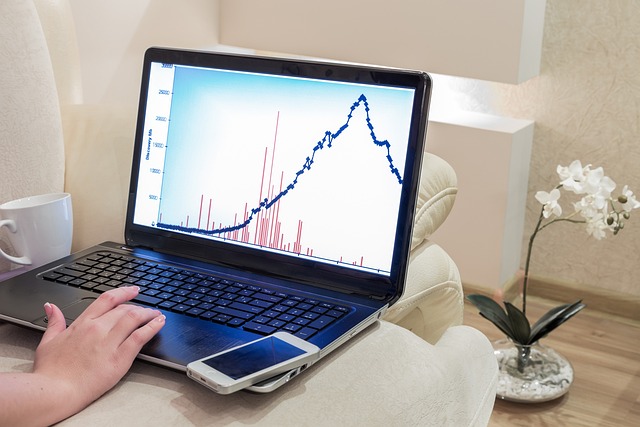
The interpretation of results is a critical stage in the data analysis process where the outputs of data models are translated into actionable insights. This step involves understanding, contextualizing, and communicating the significance of the results to inform decisions. Effective interpretation not only validates the robustness of the analytical models but also ensures that the findings can be practically applied.
Statistical Significance
- Understanding Statistical Significance: Assess the statistical significance of the findings to determine whether the observed effects are likely to be real or occurred by chance. This often involves p-values and confidence intervals.
- Threshold Setting: Establish a significance level (commonly set at 0.05) that fits the context of the analysis, where results below this threshold are considered statistically significant.
- Multiple Comparisons: Adjust for multiple comparisons if multiple hypotheses were tested simultaneously to avoid type I errors (false positives).
Business Implications
- Contextualization: Place the results within the context of the business or operational environment. Understand how these results impact current strategies, operations, or policies.
- Actionable Insights: Translate statistical results into actionable insights. Identify specific recommendations or decisions that can be made based on the findings.
- Risk Assessment: Evaluate any potential risks or uncertainties associated with the insights and suggest mitigation strategies.
Communicating Results
- Clear and Concise Reporting: Communicate the findings in a manner that is easy to understand for stakeholders, regardless of their statistical background. Use clear and concise language.
- Visualization: Employ visual aids like charts, graphs, and tables to help illustrate the findings effectively. Visual storytelling can be particularly impactful in conveying complex data.
- Discussion of Limitations: Acknowledge any limitations in the data or the analysis method that could affect the interpretation of the results.
- Feedback Incorporation: Present preliminary findings to stakeholders to gather feedback and refine the interpretation accordingly.
Operational Integration
- Implementation Strategy: Develop a strategy for how the insights will be integrated into operational processes or decision-making frameworks.
- Performance Metrics: Establish metrics to measure the impact of implementing these insights in real-world scenarios.
- Follow-up Studies: Recommend areas for further study or additional data collection that may enhance understanding or address unresolved questions.
7. Documentation and Communication
Documentation and communication are essential final steps in the data analysis process, ensuring that the methodologies, findings, and implications are clearly recorded and effectively conveyed to stakeholders. This phase is critical for transparency, reproducibility, and the effective implementation of insights derived from the data analysis.
Documentation
- Comprehensive Recording: Document every aspect of the data analysis process, from data collection and cleaning methodologies to the specifics of data modeling and interpretation of results.
- Methodological Details: Include detailed descriptions of the analytical methods and algorithms used, parameters set, and reasons for their choices. This helps in understanding the approach and facilitates reproducibility.
- Data Description: Provide a thorough description of the data, including sources, any transformations applied, and the final dataset used for analysis.
- Results Summary: Summarize the findings, including statistical significance, confidence intervals, and any other metrics that quantify the reliability of the results.
- Limitations and Assumptions: Clearly state any limitations in the data or analysis, as well as the assumptions made during the process. Discuss how these might affect the findings and their interpretation.
Communication
- Target Audience: Tailor the communication style and detail level to the audience’s expertise and interest. Technical stakeholders might require detailed methodological descriptions, whereas business stakeholders might prefer a focus on insights and actionable recommendations.
- Clarity and Simplicity: Use clear, concise language and avoid unnecessary jargon. Even complex ideas can be explained through simple explanations or analogies.
- Effective Visualizations: Employ charts, graphs, and other visual tools to illustrate the data and findings effectively. Good visuals can convey complex information quickly and clearly.
- Presentation and Reports: Develop structured reports or presentations that logically flow from objectives and data description to findings and recommendations. Provide summaries for easier digestion of key points.
- Interactive Elements: Consider using interactive dashboards or visualizations that allow users to explore data and results on their own terms. This can be particularly effective for engaging stakeholders and enabling them to understand the implications deeply.
Feedback Loop
- Stakeholder Feedback: Encourage feedback from all stakeholders to understand their perspectives, address any concerns, and clarify any confusion regarding the findings.
- Iterative Improvement: Use the feedback to refine the analysis, adjust communications, or explore additional analyses that might address unanswered questions or new issues raised by stakeholders.
About Extract Alpha
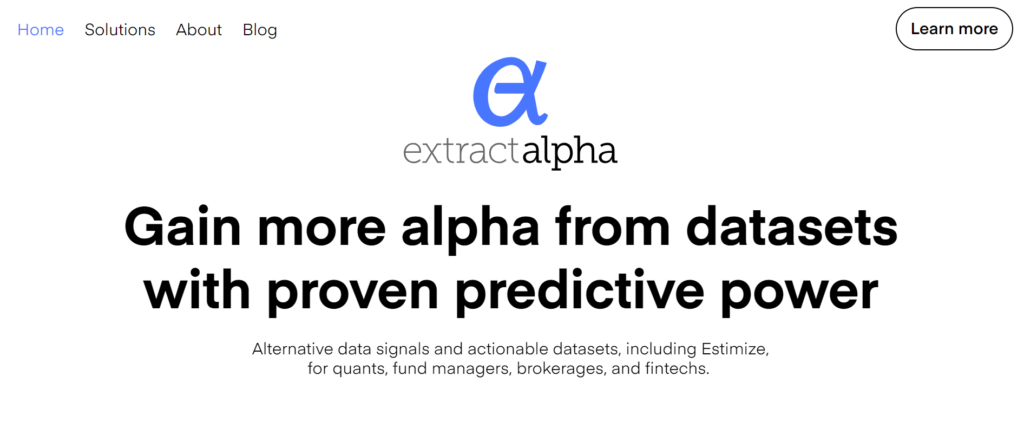
Extract Alpha is a prominent player in the financial services industry, specializing in providing advanced data analytics solutions and insights to hedge funds and asset management firms. Managing a vast array of assets totaling more than $1.5 trillion across the United States, Europe, the Middle East, Africa (EMEA), and the Asia Pacific regions, Extract Alpha stands at the forefront of financial analytics and data-driven investment strategies.
Clientele and Services
- Target Audience: Extract Alpha caters to a diverse range of clients within the financial sector, including quants, data specialists, and asset managers.
- Custom Solutions: The company offers tailored data sets and signals that are meticulously crafted to meet the unique needs and demands of its clients, enhancing their decision-making processes and investment strategies.
Innovation and Expertise
- Cutting-edge Technology: Extract Alpha is committed to using state-of-the-art technology and innovative data analysis techniques to provide the most accurate and actionable financial insights.
- Expert Team: The company employs a team of experts in data science and financial analysis, ensuring that all solutions are grounded in professional knowledge and delivered with technical proficiency.
Commitment to Excellence
- Quality Assurance: Extract Alpha places a high emphasis on the quality and reliability of its data products, implementing rigorous validation processes to ensure accuracy and consistency.
- Client Support: Superior client service is a cornerstone of their business, with a focus on providing ongoing support and advisory services to help clients navigate the complex landscape of financial investments effectively.
Strategic Impact
- Enhanced Decision-Making: By providing comprehensive data analytics, Extract Alpha empowers its clients to make informed, data-driven decisions that optimize performance and minimize risks.
- Competitive Advantage: Access to Extract Alpha’s advanced data sets and analytical tools provides clients with a competitive edge in the rapidly evolving financial markets.
Future Outlook
- Innovation Focus: Continuing to invest in research and development to stay ahead of technological advancements and market trends.
- Expansion Plans: Expanding its reach and service offerings to address emerging needs and opportunities within the financial sector.
Conclusion
Mastering the seven steps of data analysis is a journey toward extracting valuable insights from raw data. By defining objectives, collecting and preparing data meticulously, exploring dataset characteristics, preprocessing variables, applying appropriate modeling techniques, interpreting results, and effectively communicating findings, you can harness the power of data for informed decision-making.