Comparative analysis data refers to information collected and organized for the purpose of comparing and contrasting different entities, phenomena, or time periods.
This type of data is crucial for making informed decisions, identifying trends, and drawing meaningful conclusions across various fields of study and practice.
In today’s data-driven world, the ability to effectively gather, analyze, and interpret comparative data has become increasingly important.
Whether in business, science, policy-making, or academia, comparative analysis data provides the foundation for evidence-based decision-making and continuous improvement.
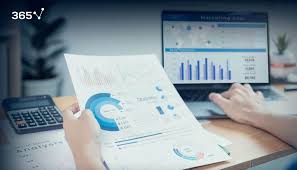
Types of Comparative Analysis Data
Quantitative data
Quantitative data consists of numerical information that can be measured and analyzed statistically. Examples include:
- Financial metrics (e.g., revenue, profit margins)
- Demographic data (e.g., population size, age distribution)
- Performance indicators (e.g., sales figures, production rates)
This type of data allows for precise comparisons and can be easily visualized through charts and graphs.
Qualitative data
Qualitative data is non-numerical and focuses on characteristics and qualities. It includes:
- Descriptive text (e.g., customer reviews, interview transcripts)
- Visual data (e.g., photographs, videos)
- Categorical data (e.g., product types, industry sectors)
While more challenging to quantify, qualitative data provides rich contextual information essential for comprehensive comparisons.
Mixed methods data
Mixed methods data combines both quantitative and qualitative elements, offering a more holistic view for comparative analysis. This approach can provide a deeper understanding of complex phenomena by leveraging the strengths of both data types.
Sources of Comparative Analysis Data
Primary sources
Primary sources are original data collected firsthand for the specific purpose of the analysis. These include:
- Surveys conducted by the researcher
- Direct observations
- Experiments designed for the study
- Original documents or records
Primary data offers the advantage of being tailored to the specific research questions but can be time-consuming and expensive to collect.
Secondary sources
Secondary sources are pre-existing data collected by others, which can be repurposed for comparative analysis. Examples include:
- Government statistics and reports
- Industry databases
- Published research studies
- Company financial reports
Secondary data is often more accessible and cost-effective but may not perfectly align with the specific needs of the analysis.
Tertiary sources
Tertiary sources compile and summarize information from primary and secondary sources. These can include:
- Textbooks
- Encyclopedias
- Literature reviews
While convenient, tertiary sources should be used cautiously in comparative analysis, as they may lack the detail and specificity required for in-depth comparisons.
Data Collection Methods
Surveys and questionnaires
Surveys and questionnaires are versatile tools for collecting both quantitative and qualitative data from large groups. They can be administered online, by phone, or in person, allowing for efficient data collection across diverse populations.
Interviews
Interviews provide in-depth qualitative data through direct conversations with subjects. They can be structured, semi-structured, or unstructured, offering flexibility in data collection based on the research needs.
Observational studies
Observational studies involve systematically watching and recording behaviors or phenomena in their natural setting. This method is particularly useful for collecting data on processes, behaviors, and interactions that may be difficult to capture through other means.
Experimental research
Experimental research involves manipulating variables in a controlled environment to observe their effects. This method is particularly valuable for establishing causal relationships and is commonly used in scientific and medical research for comparative analysis.
Database mining
Database mining involves extracting and analyzing large sets of pre-existing data. With the growth of big data, this method has become increasingly important for comparative analysis, allowing researchers to uncover patterns and trends across vast datasets.
Data Analysis Techniques
Statistical analysis
Statistical analysis uses mathematical methods to interpret quantitative data. Techniques include:
- Descriptive statistics (e.g., mean, median, standard deviation)
- Inferential statistics (e.g., t-tests, ANOVA, regression analysis)
- Multivariate analysis (e.g., factor analysis, cluster analysis)
These methods allow for rigorous comparisons and hypothesis testing.
Content analysis
Content analysis is a systematic method for categorizing and analyzing qualitative data, particularly text. It can be used to identify themes, patterns, and frequencies in large bodies of textual data, making it useful for comparing communication content across different sources or time periods.
Thematic analysis
Thematic analysis involves identifying, analyzing, and interpreting patterns or themes within qualitative data. This method is particularly useful for comparing experiences, perceptions, or beliefs across different groups or contexts.
Trend analysis
Trend analysis examines data over time to identify patterns, cycles, or directional movements. This technique is crucial for comparative analysis in fields such as economics, market research, and social sciences, where understanding temporal changes is essential.
Benchmarking
Benchmarking involves comparing performance metrics or processes against industry standards or best practices. This technique is widely used in business and organizational management to identify areas for improvement and set performance targets.
Tools and Software for Comparative Analysis
Spreadsheet software
Programs like Microsoft Excel and Google Sheets are widely used for organizing, analyzing, and visualizing comparative data. They offer a range of functions for basic statistical analysis and data manipulation.
Statistical packages
Specialized statistical software such as SPSS, SAS, and R provide advanced tools for complex statistical analyses. These packages offer a wide range of analytical techniques and are particularly useful for large datasets and sophisticated comparative studies.
Visualization tools
Data visualization tools like Tableau, Power BI, and D3.js allow for the creation of interactive and dynamic visualizations. These tools are essential for presenting comparative data in an engaging and accessible manner.
Qualitative data analysis software
Programs like NVivo, Atlas.ti, and MAXQDA facilitate the analysis of qualitative data by providing tools for coding, categorizing, and identifying patterns in textual and multimedia data.
Applications of Comparative Analysis Data
Business and market research
Comparative analysis data is crucial in business for:
- Competitive analysis
- Market trend identification
- Performance benchmarking
- Customer behavior analysis
Scientific research
In science, comparative analysis is used for:
- Evaluating treatment efficacies
- Comparing experimental results
- Analyzing species or ecosystem differences
- Studying chemical or physical property variations
Policy-making and governance
Governments and organizations use comparative data for:
- Policy impact assessment
- Cross-national comparisons
- Resource allocation decisions
- Performance evaluation of public programs
Education and academic research
In academia, comparative analysis is applied to:
- Cross-cultural studies
- Historical comparisons
- Educational system evaluations
- Literary and textual analysis
Challenges in Comparative Analysis
Data quality and reliability
Ensuring the accuracy, completeness, and consistency of data across different sources or time periods can be challenging. Poor data quality can lead to misleading comparisons and flawed conclusions.
Comparability issues
Differences in data collection methods, definitions, or measurement scales can make direct comparisons difficult. Addressing these discrepancies often requires careful data harmonization and standardization.
Cultural and contextual differences
When comparing data across different cultures or contexts, it’s crucial to consider how local factors might influence the data. Failing to account for these differences can lead to misinterpretations.
Bias and subjectivity
Researcher bias can influence data collection, analysis, and interpretation. Maintaining objectivity and acknowledging potential biases is essential for reliable comparative analysis.
Best Practices for Comparative Analysis
Defining clear objectives
Establishing clear research questions and objectives helps focus the data collection and analysis process, ensuring that the comparative analysis addresses relevant issues.
Ensuring data consistency
Standardizing data collection methods and definitions across comparison groups is crucial for meaningful analysis. When using secondary data, careful attention should be paid to understanding and aligning different data sources.
Contextualizing data
Providing adequate context for the data being compared is essential. This includes considering historical, cultural, and environmental factors that might influence the data.
Validating results
Using multiple data sources or analytical methods to cross-validate findings can increase the reliability of comparative analysis. Peer review and expert consultation can also help validate results and interpretations.
Ethical Considerations
Data privacy and protection
Ensuring the privacy and security of data, especially when dealing with sensitive or personal information, is crucial. Compliance with data protection regulations (e.g., GDPR) is essential.
Informed consent
When collecting primary data, obtaining informed consent from participants is a fundamental ethical requirement. This includes clearly explaining the purpose of the data collection and how the data will be used.
Responsible reporting of findings
Presenting comparative analysis results accurately and without bias is an ethical imperative. This includes acknowledging limitations and potential alternative interpretations of the data.
Future Trends in Comparative Analysis Data
Big data and machine learning
The integration of big data and machine learning algorithms is enabling more sophisticated and large-scale comparative analyses. These technologies allow for the identification of complex patterns and relationships across vast datasets.
Real-time comparative analysis
Advancements in data collection and processing technologies are enabling real-time comparative analysis, allowing for more dynamic and responsive decision-making in various fields.
Integration of diverse data sources
The ability to integrate and analyze data from diverse sources (e.g., IoT devices, social media, satellite imagery) is expanding the scope and depth of comparative analysis across disciplines.
Extract Alpha and Comparative Analysis Data
Extract Alpha datasets and signals are used by hedge funds and asset management firms managing more than $1.5 trillion in assets in the U.S., EMEA, and the Asia Pacific. We work with quants, data specialists, and asset managers across the financial services industry.
In the context of comparative analysis data, Extract Alpha’s expertise is particularly valuable. The company’s advanced data processing and signal generation methodologies can be applied to:
- Compare and analyze vast financial datasets across different markets, sectors, and time periods.
- Identify subtle patterns and relationships that might not be apparent through traditional comparative analysis methods.
- Generate predictive models based on comparative historical data to forecast future market trends.
- Provide benchmarks for performance comparison in various financial instruments and strategies.
- Offer insights into the relative effectiveness of different investment approaches across diverse market conditions.
As the field of comparative analysis continues to evolve, particularly in the financial sector, the sophisticated data analysis techniques employed by firms like Extract Alpha are likely to play an increasingly important role in generating actionable insights from complex, multi-dimensional datasets.
Conclusion
Comparative analysis data serves as a fundamental tool for decision-making, research, and understanding across various fields. As data collection and analysis technologies continue to advance, the potential for more sophisticated and insightful comparisons grows.
The future of comparative analysis lies in the ability to integrate diverse data sources, leverage advanced analytical techniques, and provide real-time insights. However, as the field evolves, it remains crucial to address challenges related to data quality, comparability, and ethical considerations.
By adhering to best practices and embracing emerging trends, researchers and practitioners can harness the full potential of comparative analysis data to drive innovation, improve decision-making, and deepen our understanding of complex phenomena in an increasingly interconnected world.
Commonly Asked Questions
What are the four types of comparative analysis?
The four main types of comparative analysis are:
- Descriptive Comparison: This involves describing and comparing the characteristics of different entities without making judgments about which is better.
- Normative Comparison: This type evaluates different entities against a set standard or ideal, often to determine which is “best” or most effective.
- Cause-Effect Comparison: This analysis focuses on comparing the causes and effects of different phenomena, often to understand why certain outcomes occur.
- Relational Comparison: This type examines the relationships between different variables across multiple cases or contexts.
What is an example of comparative analysis?
A common example of comparative analysis is a market comparison of smartphone brands:
- Entities compared: Apple iPhone, Samsung Galaxy, Google Pixel
- Aspects compared: Price, battery life, camera quality, operating system, storage capacity
- Data collection: Gather specifications and user reviews for each model
- Analysis: Compare each aspect across the three brands, noting similarities and differences
- Conclusion: Summarize findings, possibly recommending which phone is best for different user needs
This analysis helps consumers make informed decisions by directly comparing key features of similar products.
What statistical test is used for comparative analysis?
Several statistical tests can be used for comparative analysis, depending on the nature of the data and the specific comparison being made. Some common tests include:
- T-test: Used to compare means between two groups
- ANOVA (Analysis of Variance): Used to compare means among three or more groups
- Chi-square test: Used to compare categorical data
- Correlation analysis: Used to examine relationships between variables
- Regression analysis: Used to predict relationships between variables
- Mann-Whitney U test: A non-parametric test used when data doesn’t meet t-test assumptions
The choice of test depends on factors such as sample size, data distribution, and the type of variables being compared.
How to calculate comparative analysis?
Calculating comparative analysis typically involves these steps:
- Define the entities or variables to be compared
- Determine the metrics or criteria for comparison
- Collect relevant data for each entity
- Standardize the data if necessary (e.g., converting to percentages or ratios)
- Calculate descriptive statistics (e.g., mean, median, standard deviation) for each entity
- Perform appropriate statistical tests (e.g., t-test, ANOVA) if applicable
- Calculate percentage differences or ratios between entities
- Create visual representations (e.g., charts, graphs) to illustrate comparisons
The specific calculations will depend on the type of data and the goals of the analysis.
How to do comparative data analysis?
To conduct comparative data analysis:
- Define your research question and objectives
- Identify the entities or variables to be compared
- Select appropriate data sources and collection methods
- Gather and organize your data
- Clean and preprocess the data to ensure consistency
- Choose suitable analysis techniques (e.g., statistical tests, qualitative methods)
- Perform the analysis, comparing the entities across relevant dimensions
- Interpret the results, noting significant similarities and differences
- Validate your findings through peer review or cross-checking
- Draw conclusions and implications from your analysis
- Present your findings using clear visualizations and explanations
Remember to consider the context of your data and any limitations in your analysis.
How do you write a comparative analysis format?
A typical format for writing a comparative analysis includes:
- Introduction:
- Present the entities being compared
- State the purpose of the comparison
- Provide a thesis statement or main argument
- Background:
- Offer context for the comparison
- Explain why these entities are being compared
- Criteria for Comparison:
- List and explain the factors or aspects being compared
- Body Paragraphs:
- Organize by point-by-point or subject-by-subject method
- For each criterion or subject:
- Present data or information for each entity
- Analyze similarities and differences
- Provide evidence to support your analysis
- Discussion:
- Synthesize the comparisons made in the body
- Explain the significance of the similarities and differences
- Address any patterns or trends observed
- Conclusion:
- Summarize key findings
- Restate the main argument or insights gained from the comparison
- Discuss implications or recommendations based on the analysis
- References:
- List all sources cited in the analysis
Throughout the paper, use transitional phrases to guide the reader and maintain a balanced, objective tone while presenting your analysis.