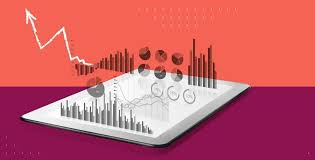
Data visualization in finance refers to the graphical representation of financial data and information.
It involves transforming complex financial datasets into visual formats that are easy to understand, analyze, and interpret.
In the fast-paced world of finance, where decisions often need to be made quickly based on large amounts of data, effective visualization has become an indispensable tool.
The importance of visual representation in financial analysis cannot be overstated. It allows financial professionals to:
- Quickly identify trends, patterns, and anomalies in data
- Communicate complex financial information more effectively
- Make data-driven decisions with greater confidence
- Present financial performance and projections in a compelling manner
As the volume and complexity of financial data continue to grow, the role of data visualization in finance is becoming increasingly crucial for both professionals and organizations.
Types of Financial Data Visualization
Charts and graphs
Traditional charts and graphs remain fundamental tools in financial data visualization:
- Line charts: Ideal for showing trends over time, such as stock price movements
- Bar charts: Effective for comparing values across categories, like revenue by product line
- Pie charts: Useful for displaying composition, such as asset allocation in a portfolio
- Scatter plots: Helpful for identifying correlations between variables, like risk and return
Heatmaps and treemaps
These visualizations are particularly useful for displaying hierarchical or multi-dimensional financial data:
- Heatmaps: Often used to show correlation matrices or trading volumes across markets
- Treemaps: Effective for visualizing hierarchical structures, such as market capitalization of stocks in different sectors
Dashboards
Financial dashboards combine multiple visualizations to provide a comprehensive view of financial performance:
- KPI dashboards: Display key performance indicators for quick assessment
- Risk dashboards: Visualize various risk metrics and exposures
- Investment dashboards: Show portfolio performance and allocation
Interactive visualizations
Modern financial visualizations often incorporate interactivity, allowing users to:
- Drill down into specific data points
- Adjust time frames or parameters
- Toggle between different metrics or views
These interactive elements enhance the depth of analysis and allow for more personalized exploration of financial data.
Key Financial Metrics for Visualization
Revenue and profit trends
Visualizations of revenue and profit trends typically include:
- Year-over-year growth charts
- Margin analysis graphs
- Revenue breakdown by product, region, or customer segment
Cash flow analysis
Cash flow visualizations often feature:
- Waterfall charts showing cash inflows and outflows
- Trend lines of operating, investing, and financing cash flows
- Forecasted vs. actual cash position graphs
Market performance indicators
Key market performance visualizations include:
- Stock price and volume charts
- Benchmark comparison graphs
- Market sentiment indicators
Risk assessments
Risk-related visualizations often encompass:
- Value at Risk (VaR) charts
- Sensitivity analysis heatmaps
- Stress test scenario comparisons
Tools for Financial Data Visualization
Spreadsheet software
Microsoft Excel and Google Sheets remain popular for basic financial visualizations due to their accessibility and familiarity. They offer a range of built-in chart types and customization options.
Specialized financial visualization software
Tools like Bloomberg Terminal, Tableau, and Power BI provide advanced visualization capabilities tailored for financial data, including real-time data integration and interactive dashboards.
Business intelligence platforms
Platforms such as Looker, Sisense, and Domo offer comprehensive solutions for financial data analysis and visualization, often with AI-powered insights and collaborative features.
Programming languages for custom visualizations
For more complex or customized visualizations, financial professionals often turn to programming languages:
- Python with libraries like Matplotlib, Seaborn, and Plotly
- R with ggplot2 and other visualization packages
- JavaScript with D3.js for web-based interactive visualizations
Best Practices in Financial Data Visualization
Choosing the right visualization type
Select the most appropriate chart type based on the data and the story you want to tell. For example:
- Use line charts for time series data
- Opt for bar charts when comparing categories
- Consider scatter plots for showing relationships between variables
Ensuring data accuracy and integrity
Maintain rigorous data quality controls to ensure the accuracy of visualizations:
- Regularly audit and clean data sources
- Implement data validation checks
- Clearly label data sources and time frames
Designing for clarity and impact
Create visualizations that are easy to understand and interpret:
- Use consistent color schemes and layouts
- Avoid clutter and unnecessary elements
- Highlight key information and insights
Tailoring visualizations to the audience
Adapt your visualizations to the needs and expertise of your audience:
- Use more detailed visualizations for technical audiences
- Simplify and focus on key messages for executive presentations
- Provide context and explanations for non-financial audiences
Applications in Different Finance Sectors
Investment banking and trading
In investment banking and trading, data visualizations are used for:
- Market trend analysis and forecasting
- Risk management and exposure monitoring
- Portfolio performance tracking
- Deal flow and pipeline visualization
Corporate finance
Corporate finance applications include:
- Financial statement analysis and reporting
- Budget vs. actual comparisons
- Capital structure optimization
- Merger and acquisition scenario modeling
Personal finance and wealth management
In personal finance and wealth management, visualizations help with:
- Asset allocation and portfolio diversification
- Retirement planning and goal tracking
- Expense analysis and budgeting
- Investment performance comparison
Regulatory reporting and compliance
Data visualization plays a crucial role in:
- Stress testing and scenario analysis
- Regulatory capital and liquidity reporting
- Anti-money laundering (AML) and fraud detection
- Environmental, Social, and Governance (ESG) reporting
Challenges in Financial Data Visualization
Handling large and complex datasets
Financial datasets are often massive and multi-dimensional, presenting challenges in:
- Data processing and aggregation
- Real-time visualization of streaming data
- Balancing detail with overview in visualizations
Ensuring data security and privacy
Given the sensitive nature of financial data, visualization tools must:
- Implement robust security measures
- Comply with data protection regulations
- Manage access controls and data anonymization
Keeping visualizations up-to-date
In the fast-moving financial world, visualizations need to:
- Reflect real-time or near-real-time data
- Automatically update with new information
- Maintain historical context while showing current trends
Avoiding misinterpretation of visual data
Challenges include:
- Preventing misleading scales or comparisons
- Clearly communicating data limitations and assumptions
- Providing necessary context for accurate interpretation
Emerging Trends in Financial Data Visualization
Real-time data visualization
Advancements in technology are enabling:
- Live streaming of financial data visualizations
- Real-time updates of dashboards and reports
- Instant visual alerts for market movements or risk thresholds
Artificial intelligence and machine learning integration
AI and ML are enhancing financial visualizations through:
- Automated pattern recognition and anomaly detection
- Predictive visualizations based on historical data
- Natural language generation to explain visual insights
Virtual and augmented reality in financial visualization
Emerging applications include:
- VR trading floors for immersive market analysis
- AR overlays of financial data in real-world contexts
- 3D visualizations of complex financial structures
Mobile-first visualization designs
With the increase in mobile usage, there’s a trend towards:
- Responsive design for various screen sizes
- Touch-friendly interactive visualizations
- Simplified views optimized for mobile devices
Impact of Data Visualization on Financial Decision-Making
Enhancing pattern recognition
Visualizations help financial professionals:
- Identify trends and cycles more quickly
- Spot outliers and anomalies in large datasets
- Recognize correlations between different financial variables
Facilitating faster decision-making
By presenting data visually, decision-makers can:
- Grasp complex financial situations more quickly
- Compare multiple scenarios side-by-side
- React more swiftly to market changes or opportunities
Improving communication of financial insights
Visual representations allow for:
- More effective presentation of financial data to stakeholders
- Clearer explanation of complex financial concepts
- Better alignment of teams around financial goals and performance
Supporting data-driven strategies
Data visualization supports strategic decision-making by:
- Providing a clear picture of current financial status
- Illustrating potential outcomes of different strategies
- Tracking progress towards financial objectives
Ethical Considerations in Financial Data Visualization
Transparency in data representation
Ethical considerations include:
- Clearly stating data sources and methodologies
- Disclosing any data manipulations or adjustments
- Providing access to underlying data when appropriate
Avoiding misleading visualizations
It’s crucial to:
- Use appropriate scales and axes
- Avoid cherry-picking data to support a particular narrative
- Present a balanced view, including both positive and negative aspects
Addressing biases in data and visualization
Efforts should be made to:
- Recognize and mitigate inherent biases in financial data
- Design visualizations that are inclusive and accessible
- Consider diverse perspectives in data interpretation
Extract Alpha and Financial Data Visualization
Extract Alpha datasets and signals are used by hedge funds and asset management firms managing more than $1.5 trillion in assets in the U.S., EMEA, and the Asia Pacific. We work with quants, data specialists, and asset managers across the financial services industry.
In the context of financial data visualization, Extract Alpha’s expertise is particularly valuable. The company’s advanced data processing and signal generation methodologies can be applied to:
- Create visually compelling representations of complex financial datasets
- Develop interactive dashboards that allow for deep exploration of market trends and anomalies
- Generate real-time visualizations of alpha signals and market indicators
- Design custom visualization tools that integrate with existing financial analysis platforms
- Provide visual representations of backtested strategies and performance metrics
As the field of financial data visualization continues to evolve, the sophisticated data analysis techniques employed by firms like Extract Alpha are likely to play an increasingly important role in translating complex financial data into actionable visual insights.
Conclusion
The future of data visualization in finance is bright, with continued advancements in technology and data analytics promising even more sophisticated and insightful visual representations of financial information. As financial data becomes increasingly complex and voluminous, the ability to effectively visualize this data will be a key differentiator for financial professionals and organizations.
Key takeaways for financial professionals:
- Invest in developing strong data visualization skills
- Stay updated on the latest visualization tools and techniques
- Always consider the audience and purpose when creating visualizations
- Maintain ethical standards in data representation
- Leverage visualizations to enhance decision-making and communication
As the finance industry continues to evolve, those who can harness the power of data visualization will be well-positioned to navigate the complexities of the financial world and drive informed, data-driven decisions.
Frequently Asked Questions
How is data visualization used in finance?
Data visualization in finance is used to:
- Analyze market trends and patterns
- Monitor portfolio performance
- Assess risk factors and exposures
- Present financial reports and key performance indicators (KPIs)
- Forecast financial outcomes and scenarios
- Detect anomalies or fraudulent activities
- Communicate complex financial information to stakeholders
- Support investment decision-making processes
- Track budget allocations and expenditures
- Analyze customer behavior and preferences in financial services
What is the best visualization for finance?
The best visualization depends on the specific financial data and the story you want to tell. However, some widely used and effective visualizations in finance include:
- Line charts: For tracking stock prices, economic indicators, or performance over time
- Bar charts: For comparing financial metrics across categories or time periods
- Heatmaps: For visualizing correlations or risk exposures
- Candlestick charts: For detailed stock price analysis
- Treemaps: For hierarchical data like market capitalization across sectors
- Scatter plots: For showing relationships between financial variables
- Dashboards: For comprehensive views of multiple financial metrics
The key is to choose a visualization that clearly and accurately represents your data and insights.
What is data visualization in banking?
Data visualization in banking refers to the graphical representation of banking-related data to improve understanding and decision-making. It is used for:
- Customer segmentation and behavior analysis
- Risk management and fraud detection
- Branch performance monitoring
- Loan and deposit portfolio analysis
- Regulatory compliance reporting
- Market trend analysis for new product development
- Operational efficiency monitoring
- Credit scoring and loan approval processes
- Asset and liability management
- Customer experience and satisfaction tracking
Banks use various visualization tools to transform complex financial data into intuitive visual formats, helping them to identify trends, mitigate risks, and improve services.
How is data analytics used in finance?
Data analytics in finance is used to:
- Predict market trends and asset prices
- Assess and manage financial risks
- Detect fraudulent transactions
- Optimize investment portfolios
- Automate trading strategies (algorithmic trading)
- Personalize financial products and services
- Improve credit scoring models
- Enhance regulatory compliance and reporting
- Analyze customer churn and retention in financial services
- Forecast cash flows and financial performance
- Optimize pricing strategies for financial products
- Conduct sentiment analysis for market predictions
These applications help financial institutions make data-driven decisions, improve operational efficiency, and gain competitive advantages.
What are the 4 types of data analytics?
The four main types of data analytics are:
- Descriptive Analytics: Summarizes what has happened in the past. It uses historical data to identify patterns and relationships. In finance, this could involve analyzing past stock performance or financial statements.
- Diagnostic Analytics: Focuses on why something happened. It involves deeper data exploration to understand the causes of past performance or events. In finance, this might include analyzing why a particular investment strategy failed or succeeded.
- Predictive Analytics: Forecasts what is likely to happen in the future. It uses historical data and statistical modeling to predict future trends. In finance, this could involve predicting stock prices, market trends, or customer behaviors.
- Prescriptive Analytics: Suggests actions to take based on the insights gained. It combines the insights from the other types of analytics to recommend optimal courses of action. In finance, this might include recommending investment strategies or risk management approaches.
How do you analyse data in finance?
Analyzing data in finance typically involves the following steps:
- Define objectives: Clearly outline what you want to achieve with the analysis.
- Collect data: Gather relevant financial data from various sources (e.g., financial statements, market data, economic indicators).
- Clean and prepare data: Ensure data accuracy, handle missing values, and format data consistently.
- Exploratory data analysis: Use descriptive statistics and visualizations to understand the basic features of the data.
- Apply analytical techniques: Use appropriate methods such as:
- Ratio analysis for assessing financial health
- Time series analysis for identifying trends
- Regression analysis for understanding relationships between variables
- Risk modeling for assessing potential losses
- Use financial models: Apply models like Discounted Cash Flow (DCF) or Capital Asset Pricing Model (CAPM) as appropriate.
- Leverage technology: Utilize financial software, data visualization tools, and programming languages like Python or R for complex analyses.
- Interpret results: Draw meaningful conclusions from the analysis.
- Validate findings: Cross-check results and consider alternative interpretations.
- Communicate insights: Present findings clearly, often using data visualizations to support your conclusions.
- Iterate and refine: Continuously refine your analysis based on new data and feedback.
Remember, the specific approach will vary depending on the particular financial question or problem you’re addressing.