In the age of big data, the ability to compare and contrast different data sets is a crucial skill for researchers, analysts, and decision-makers across various fields.
Whether you’re comparing clinical trial results, analyzing market trends, or evaluating environmental changes, understanding the statistical methods for comparing two data sets is essential.
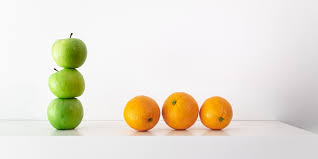
Importance of comparing data sets
Comparing data sets allows us to draw meaningful conclusions, identify patterns, and make informed decisions. It helps us answer questions like:
- Are there significant differences between two groups?
- Has a particular intervention had a measurable effect?
- Are two variables related, and if so, how strongly?
By applying rigorous statistical methods, we can move beyond anecdotal evidence and gut feelings to make data-driven decisions. This is particularly important in fields such as medicine, where treatment efficacy must be scientifically proven, or in business, where understanding customer behavior can lead to strategic advantages.
Overview of statistical comparison methods
Statistical comparison methods range from simple descriptive statistics to complex multivariate analyses. The choice of method depends on the nature of your data, your research questions, and the assumptions you can make about your data. In this article, we’ll explore various techniques, from basic to advanced, that can help you compare two data sets effectively.
These methods have evolved over time, from early techniques developed in the early 20th century to modern computational approaches that can handle large and complex data sets. Understanding this range of tools allows analysts to choose the most appropriate method for their specific needs.
Preparing Data for Comparison
Before diving into statistical analyses, it’s crucial to ensure your data is clean, consistent, and properly formatted. This preparatory phase often takes more time than the analysis itself but is vital for obtaining reliable results.
Ensuring data quality and consistency
Start by checking for data entry errors, inconsistent formatting, and duplicate entries. Ensure that your variables are correctly coded and that categorical variables are consistent across both data sets. For instance, if you’re comparing survey results, make sure that response options are coded identically in both sets.
Data quality checks might include:
- Looking for impossible values (e.g., negative ages)
- Checking for consistency in units of measurement
- Verifying that categorical variables use the same coding scheme across data sets
Handling missing values and outliers
Missing data can significantly impact your analysis. Decide on a strategy for dealing with missing values, such as:
- Listwise deletion (removing cases with any missing data)
- Pairwise deletion (using all available data for each analysis)
- Imputation (estimating missing values based on other available data)
Each method has its pros and cons. Listwise deletion is simple but can dramatically reduce your sample size. Imputation methods like multiple imputation can preserve sample size but require careful implementation to avoid biasing your results.
Outliers can also skew your results. Identify outliers using methods like the Interquartile Range (IQR) or z-scores, and decide whether to:
- Remove them if they’re due to errors
- Transform them to reduce their impact
- Use robust statistical methods that are less sensitive to outliers
The decision should be based on your understanding of the data and the research context.
Normalization and standardization techniques
If your data sets use different scales or units, you may need to normalize or standardize them before comparison. Common techniques include:
- Min-max scaling: Scaling values to a fixed range, typically 0 to 1
- Z-score standardization: Transforming data to have a mean of 0 and a standard deviation of 1
- Log transformation: Useful for right-skewed data or when dealing with ratios
For example, if you’re comparing salaries across different countries, you might need to standardize the values to account for differences in currency and cost of living.
Descriptive Statistics
Descriptive statistics provide a summary of your data sets and can highlight initial differences or similarities. They’re often the first step in any data analysis project.
Measures of central tendency
- Mean: The average of all values in a data set
- Median: The middle value when data is ordered
- Mode: The most frequent value in the data set
Calculate these for both data sets to get an initial sense of how they differ. For example, if you’re comparing salaries in two companies, you might find that Company A has a higher mean salary but a lower median, suggesting a few high earners skewing the average.
The choice between mean, median, and mode depends on your data distribution. For skewed data, the median is often more informative than the mean.
Measures of variability
- Range: The difference between the maximum and minimum values
- Variance: The average squared deviation from the mean
- Standard deviation: The square root of the variance, providing a measure of spread in the same units as the original data
- Interquartile range (IQR): The range between the 25th and 75th percentiles
These measures help you understand how spread out your data is. A data set with a larger standard deviation indicates more variability in the data.
For example, when comparing test scores between two classes, you might find that while the means are similar, one class has a much larger standard deviation, indicating more diverse performance levels.
Visualization techniques
Visual representations can provide intuitive comparisons between data sets:
- Histograms: Show the distribution of continuous variables
- Box plots: Display the median, quartiles, and potential outliers
- Scatter plots: Useful for examining relationships between two continuous variables
- Q-Q plots: Help assess whether data follows a particular distribution (e.g., normal distribution)
For instance, overlaying histograms of test scores from two classes can quickly show differences in distribution and central tendency. Box plots can effectively compare multiple groups side by side, showing not just central tendencies but also spread and potential outliers.
Hypothesis Testing
Hypothesis testing forms the backbone of inferential statistics, allowing us to make claims about populations based on sample data.
Null and alternative hypotheses
The null hypothesis (H0) typically assumes no difference or no effect, while the alternative hypothesis (H1) suggests a difference or effect exists. For example: H0: There is no difference in mean test scores between two teaching methods H1: There is a difference in mean test scores between two teaching methods
It’s crucial to formulate clear, testable hypotheses before conducting your analysis. This helps prevent p-hacking (the practice of manipulating analyses to find statistically significant results).
Significance levels and p-values
The significance level (α) is the probability of rejecting the null hypothesis when it’s actually true. Common values are 0.05 and 0.01. The p-value is the probability of obtaining results at least as extreme as those observed, assuming the null hypothesis is true. If the p-value is less than α, we reject the null hypothesis.
It’s important to note that the choice of significance level is somewhat arbitrary and should be made before conducting the analysis. Some fields are moving towards using lower α values (e.g., 0.005) to reduce false positives.
Type I and Type II errors
- Type I error: Rejecting the null hypothesis when it’s actually true (false positive)
- Type II error: Failing to reject the null hypothesis when it’s actually false (false negative)
Understanding these errors is crucial for interpreting your results and assessing the reliability of your conclusions. The probability of a Type II error is denoted as β, and statistical power is defined as 1 – β.
There’s often a trade-off between Type I and Type II errors. Lowering the significance level (α) reduces the chance of Type I errors but increases the chance of Type II errors.
Parametric Tests for Comparing Two Data Sets
Parametric tests assume that your data follows a particular distribution, typically the normal distribution. They are generally more powerful than non-parametric tests when their assumptions are met.
Independent samples t-test
Use this test when comparing the means of two independent groups. For example, comparing test scores between two different schools. The test assumes:
- Independent observations
- Normally distributed data
- Homogeneity of variances
The t-test is widely used and relatively robust to minor violations of its assumptions, especially with large sample sizes.
Paired samples t-test
This test is used when you have two measurements on the same subjects, such as before and after an intervention. It assumes:
- Paired observations
- Normally distributed differences between pairs
The paired t-test is often more powerful than the independent t-test when you have matched pairs, as it accounts for the correlation between measurements.
Analysis of Variance (ANOVA)
While primarily used for comparing more than two groups, ANOVA can be used to compare two groups as well. It’s particularly useful when you want to control for other variables. One-way ANOVA for two groups will give the same results as an independent samples t-test.
ANOVA can be extended to more complex designs, such as:
- Two-way ANOVA: Examining the effect of two factors simultaneously
- Repeated measures ANOVA: For multiple measurements on the same subjects over time
Non-parametric Tests for Comparing Two Data Sets
When your data doesn’t meet the assumptions for parametric tests, non-parametric alternatives are available. These tests are often based on ranks rather than the actual values of the data.
Mann-Whitney U test
This is the non-parametric alternative to the independent samples t-test. It compares the distributions of two independent groups and is particularly useful for ordinal data or when you can’t assume normality.
The test statistic U is calculated by ranking all the values from both groups together, then summing the ranks for one group. The null hypothesis is that the probability of an observation from one population exceeding an observation from the second population is equal to the probability of an observation from the second population exceeding an observation from the first population.
Wilcoxon signed-rank test
This is the non-parametric version of the paired samples t-test. It’s used for comparing two related samples or repeated measurements on a single sample.
The test involves calculating the differences between pairs of observations, ranking these differences, and then comparing the sum of ranks for positive and negative differences.
Kruskal-Wallis test
While typically used for comparing more than two groups, this test can be used for two groups as an alternative to one-way ANOVA when the assumptions of ANOVA are not met.
For two groups, the Kruskal-Wallis test gives the same results as the Mann-Whitney U test.
Correlation Analysis
Correlation analysis helps determine the strength and direction of the relationship between two variables.
Pearson correlation coefficient
This measures the strength of the linear relationship between two continuous variables. It ranges from -1 to 1, where:
- 1 indicates a perfect positive linear relationship
- 1 indicates a perfect negative linear relationship
- 0 indicates no linear relationship
Pearson’s correlation assumes that both variables are normally distributed and that there is a linear relationship between them.
Spearman’s rank correlation coefficient
This non-parametric measure is used when you can’t assume a linear relationship or when dealing with ordinal variables. It measures the strength and direction of the monotonic relationship between two variables.
Spearman’s correlation is calculated using the same formula as Pearson’s correlation, but on the ranks of the data rather than the actual values.
Interpreting correlation results
Remember that correlation doesn’t imply causation. A strong correlation might suggest a relationship, but other factors could be at play. Always consider potential confounding variables and the context of your data.
Guidelines for interpreting correlation strength:
- 0.00-0.19: Very weak
- 0.20-0.39: Weak
- 0.40-0.59: Moderate
- 0.60-0.79: Strong
- 0.80-1.0: Very strong
However, these are just rules of thumb and the practical significance of a correlation depends on the context of your research.
Effect Size and Power Analysis
While statistical significance tells us whether an effect is likely to exist in the population, effect size tells us how large that effect is.
Cohen’s d
Cohen’s d is a measure of effect size that expresses the difference between two group means in terms of standard deviations. It helps you understand the practical significance of your results, not just statistical significance.
Interpreting Cohen’s d:
- 0.2: Small effect
- 0.5: Medium effect
- 0.8: Large effect
Statistical power
Power is the probability of correctly rejecting the null hypothesis when it’s false. A power of 0.8 or higher is generally considered good. Factors affecting power include:
- Sample size
- Effect size
- Significance level
Power analysis can be conducted a priori (before the study) to determine the required sample size, or post hoc (after the study) to interpret non-significant results.
Sample size considerations
Larger sample sizes increase statistical power but may be costly or impractical. Power analysis can help you determine the minimum sample size needed to detect an effect of a given size with a certain level of confidence.
For example, to detect a medium effect size (d = 0.5) with 80% power at α = 0.05 for an independent samples t-test, you would need approximately 64 participants per group.
Advanced Techniques
As data sets become larger and more complex, more sophisticated techniques are often needed for comparison.
Multivariate analysis
When dealing with multiple variables, techniques like MANOVA (Multivariate Analysis of Variance) or discriminant analysis can be useful for comparing groups across several dependent variables simultaneously.
MANOVA extends the concepts of ANOVA to situations where you have multiple dependent variables. It can help control for Type I error inflation that would occur if you ran multiple separate ANOVAs.
Machine learning approaches for data set comparison
Machine learning techniques like clustering algorithms (e.g., k-means) or dimensionality reduction methods (e.g., principal component analysis) can be powerful tools for comparing complex, high-dimensional data sets.
For example, t-SNE (t-distributed stochastic neighbor embedding) can be used to visualize high-dimensional data sets in two or three dimensions, allowing for visual comparison.
Bayesian methods
Bayesian approaches offer an alternative framework for comparing data sets. They allow for the incorporation of prior knowledge and can be particularly useful when dealing with small sample sizes or complex models.
Bayesian methods provide probabilistic statements about parameters, rather than binary decisions based on p-values. This can lead to more nuanced interpretations of data.
Practical Considerations
Choosing the appropriate test
The choice of test depends on various factors:
- Type of data (continuous, categorical, ordinal)
- Distribution of data (normal or non-normal)
- Independence of observations
- Research question (comparing means, distributions, relationships)
Always check the assumptions of your chosen test and consider alternatives if these assumptions are violated. It’s often helpful to create a decision tree to guide your choice of statistical test based on your data characteristics and research question.
Assumptions and limitations of different methods
Each statistical method comes with its own set of assumptions and limitations. Common assumptions include:
- Normality of data
- Homogeneity of variances
- Independence of observations
- Random sampling
Violating these assumptions can lead to incorrect conclusions, so it’s crucial to understand and check them for your chosen method. Techniques like Q-Q plots, Levene’s test for homogeneity of variances, and the Shapiro-Wilk test for normality can help assess these assumptions.
Interpreting and reporting results
When reporting results:
- Clearly state your hypotheses
- Describe your methods, including any data preprocessing steps
- Report relevant statistics (e.g., test statistic, degrees of freedom, p-value)
- Include measures of effect size
- Discuss the practical significance of your findings, not just statistical significance
- Be transparent about any limitations or potential sources of bias in your study
Remember to report your results in a way that is accessible to your intended audience. This might involve creating clear visualizations or explaining statistical concepts in layman’s terms.
Case Studies
Comparing clinical trial results
In a hypothetical study comparing two treatments for hypertension, researchers might use an independent samples t-test to compare mean blood pressure reduction between the two groups. They would also consider factors like sample size, effect size, and potential confounding variables.
Analyzing market research data
A company comparing customer satisfaction scores between two product lines might use a Mann-Whitney U test if the satisfaction scores are ordinal. They could also examine the correlation between satisfaction scores and purchase frequency using Spearman’s rank correlation.
Environmental data comparison
Researchers comparing pollution levels in two cities over time might use a paired samples t-test if measurements were taken at the same times in both cities. They would need to consider seasonal variations and potential outliers due to unusual events.
Extract Alpha and Financial Data Analysis
Extract Alpha datasets and signals are used by hedge funds and asset management firms managing more than $1.5 trillion in assets in the U.S., EMEA, and the Asia Pacific. We work with quants, data specialists, and asset managers across the financial services industry.
In the context of financial data analysis, the statistical comparison of two data sets becomes particularly crucial. Hedge funds and asset management firms often need to compare different investment strategies, market performance across regions, or the effectiveness of various predictive models. The techniques discussed in this article, from basic descriptive statistics to advanced machine learning approaches, are all applicable in the financial sector.
For instance, a fund manager might use paired t-tests to compare the performance of their portfolio against a benchmark index over time. Correlation analysis could be employed to understand the relationships between different economic indicators and stock prices. Advanced techniques like multivariate analysis might be used to compare the performance of multiple assets simultaneously.
The large-scale adoption of Extract Alpha’s datasets and signals by firms managing such significant assets underscores the importance of robust, reliable statistical comparisons in the financial world. As the complexity and volume of financial data continue to grow, the demand for sophisticated statistical analysis techniques is likely to increase correspondingly.
Conclusion
Statistical comparison of two data sets is a powerful tool for drawing insights and making decisions. From basic descriptive statistics to advanced multivariate techniques, the choice of method depends on your data and research questions.