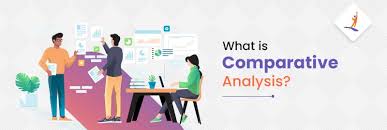
Comparative analysis statistics refers to the set of statistical methods and techniques used to compare and contrast different groups, datasets, or phenomena.
This approach is fundamental in research and decision-making across various fields, allowing for the systematic evaluation of similarities, differences, and relationships between different entities or over time.
The importance of comparative analysis in modern research and business cannot be overstated. It provides a structured way to test hypotheses, evaluate the effectiveness of interventions, and make data-driven decisions. By employing rigorous statistical methods, comparative analysis helps to move beyond anecdotal evidence and subjective impressions, offering a more objective basis for conclusions and actions.
Types of Comparative Analysis
Descriptive comparisons
These involve comparing basic statistical measures (such as means, medians, and standard deviations) between groups. Descriptive comparisons provide a fundamental understanding of how datasets differ in their central tendencies and variability.
Inferential comparisons
Inferential statistics allow researchers to draw conclusions about populations based on sample data. These comparisons involve hypothesis testing and estimation, enabling generalizations beyond the immediate data.
Longitudinal comparisons
These analyze changes over time within the same group or entity. Longitudinal studies are crucial for understanding trends, developmental processes, and the effects of interventions over extended periods.
Cross-sectional comparisons
Cross-sectional analyses compare different groups at a single point in time. This approach is useful for understanding how various factors or characteristics differ across populations.
Key Statistical Methods
T-tests
T-tests are used to compare means between two groups. There are several types:
- Independent samples t-test: Compares means between two unrelated groups
- Paired samples t-test: Compares means between two related measurements
- One-sample t-test: Compares a sample mean to a known population mean
Analysis of Variance (ANOVA)
ANOVA extends the concept of t-tests to comparisons involving three or more groups. It includes:
- One-way ANOVA: Compares means across multiple groups with one independent variable
- Two-way ANOVA: Examines the effect of two independent variables on a dependent variable
- Repeated measures ANOVA: Analyzes data from the same subjects measured multiple times
Chi-square tests
Chi-square tests are used for categorical data, assessing whether observed frequencies differ significantly from expected frequencies. They’re particularly useful in analyzing the association between categorical variables.
Regression analysis
Regression techniques examine the relationship between one or more independent variables and a dependent variable. Key types include:
- Simple linear regression
- Multiple regression
- Logistic regression for binary outcomes
Non-parametric tests
These tests are used when data doesn’t meet the assumptions of parametric tests (like normal distribution). Examples include:
- Mann-Whitney U test (alternative to independent t-test)
- Wilcoxon signed-rank test (alternative to paired t-test)
- Kruskal-Wallis test (alternative to one-way ANOVA)
Data Collection and Preparation
Sampling techniques
Proper sampling is crucial for valid comparative analysis. Techniques include:
- Random sampling
- Stratified sampling
- Cluster sampling
- Convenience sampling (with noted limitations)
Data cleaning and normalization
Ensuring data quality through cleaning and normalization is essential. This includes:
- Removing or correcting erroneous entries
- Standardizing data formats
- Normalizing scales for fair comparisons
Handling missing data
Strategies for dealing with missing data include:
- Listwise deletion
- Pairwise deletion
- Imputation methods (mean imputation, regression imputation, multiple imputation)
Ensuring data comparability
When comparing different datasets, it’s crucial to ensure they are truly comparable. This may involve:
- Aligning definitions and measures
- Adjusting for differences in population characteristics
- Considering contextual factors that might affect comparability
Visualization Techniques
Comparative bar charts and histograms
These visualizations are effective for comparing distributions and frequencies across groups.
Box plots and whisker diagrams
Box plots provide a concise display of the distribution of a variable, showing median, quartiles, and potential outliers.
Scatter plots for correlation analysis
Scatter plots visualize the relationship between two continuous variables, useful for identifying patterns and correlations.
Heat maps for multivariable comparisons
Heat maps can display complex, multivariable comparisons, using color intensity to represent values across multiple dimensions.
Interpreting Results
Statistical significance
Understanding p-values and their limitations is crucial. A statistically significant result suggests that the observed difference is unlikely to have occurred by chance.
Effect size
Effect size measures the magnitude of the difference between groups, providing context to statistical significance.
Confidence intervals
Confidence intervals offer a range of plausible values for population parameters, providing more information than point estimates alone.
Practical vs. statistical significance
It’s important to distinguish between statistical significance and practical importance. A statistically significant result may not always be meaningful in real-world terms.
Common Pitfalls and Challenges
Selection bias
Biased sampling can lead to non-representative results. Researchers must be aware of potential sources of bias in their sampling methods.
Confounding variables
Unaccounted-for variables can influence the relationship between the variables of interest, leading to spurious correlations or masking true effects.
Multiple comparisons problem
When conducting multiple statistical tests, the chance of Type I errors (false positives) increases. Techniques like Bonferroni correction can address this issue.
Assumption violations in statistical tests
Many statistical tests have underlying assumptions (e.g., normality, homogeneity of variance). Violating these assumptions can lead to invalid results.
Applications in Various Fields
Business and market research
Comparative analysis is used in market segmentation, product comparisons, and competitive analysis.
Scientific research
In fields like biology, physics, and chemistry, comparative analysis is crucial for hypothesis testing and understanding complex phenomena.
Social sciences
Sociologists, psychologists, and economists use comparative methods to study human behavior, societal trends, and economic phenomena.
Healthcare and clinical trials
Comparative analysis is fundamental in evaluating treatment efficacy, comparing patient outcomes, and conducting epidemiological studies.
Advanced Techniques
Multivariate analysis
Techniques like MANOVA and discriminant analysis allow for the simultaneous comparison of multiple dependent variables.
Meta-analysis
Meta-analysis combines results from multiple studies to provide a comprehensive view of a research question.
Bayesian comparative methods
Bayesian approaches offer an alternative framework for comparative analysis, incorporating prior knowledge and updating beliefs based on new data.
Machine learning in comparative analysis
Machine learning algorithms can identify patterns and relationships in large, complex datasets, enhancing traditional comparative techniques.
Ethical Considerations
Transparency in methodology
Researchers should clearly report their methods, including any data transformations or exclusions.
Responsible reporting of results
Results should be reported fully and accurately, including negative or inconclusive findings.
Avoiding cherry-picking data
Selectively reporting only favorable results undermines the integrity of comparative analysis.
Software Tools for Comparative Analysis
Statistical packages
Popular tools include SPSS, SAS, and R, offering a wide range of statistical functions and customization options.
Data visualization tools
Software like Tableau and Power BI provide powerful visualization capabilities for comparative data.
Specialized research software
Field-specific software packages often include tailored comparative analysis features.
Future Trends
Big data and comparative analysis
The increasing availability of large datasets is enabling more comprehensive and nuanced comparative analyses.
AI-driven comparative studies
Artificial intelligence and machine learning are enhancing the ability to identify complex patterns and relationships in comparative data.
Real-time comparative analytics
Advances in data processing are enabling real-time comparative analysis, particularly valuable in fast-moving fields like finance and healthcare.
Extract Alpha and Comparative Analysis Statistics
Extract Alpha datasets and signals are used by hedge funds and asset management firms managing more than $1.5 trillion in assets in the U.S., EMEA, and the Asia Pacific. We work with quants, data specialists, and asset managers across the financial services industry.
In the context of comparative analysis statistics, Extract Alpha’s expertise can be particularly valuable. The company’s advanced data processing and signal generation methodologies can be applied to:
- Conduct sophisticated comparative analyses of financial instruments across different markets and time periods
- Develop custom benchmarks for performance comparison in various investment strategies
- Identify statistically significant anomalies or patterns in financial data through comparative techniques
- Provide insights into the relative effectiveness of different investment approaches under varying market conditions
- Offer tools for real-time comparative analysis of market trends and investor behavior
As the field of comparative analysis continues to evolve, especially in the financial sector, the sophisticated statistical techniques employed by firms like Extract Alpha are likely to play an increasingly important role in generating actionable insights from complex, multi-dimensional datasets.
Conclusion
Comparative analysis statistics remain a cornerstone of empirical research and data-driven decision-making across numerous fields. The ability to systematically compare and contrast different groups, interventions, or time periods provides invaluable insights that drive progress in science, business, and policy.
As we move forward, the challenge lies in balancing the rigorous application of statistical methods with the need for practical, actionable insights. The increasing complexity of data and the advent of new analytical tools offer exciting opportunities for more nuanced and comprehensive comparisons. However, it’s crucial to maintain a focus on interpretability and real-world relevance.
The future of comparative analysis will likely see a greater integration of traditional statistical methods with advanced machine learning techniques, allowing for more sophisticated handling of large and complex datasets. At the same time, there will be an ongoing need for careful consideration of ethical issues, particularly around data privacy and the responsible reporting of results.
Ultimately, the power of comparative analysis lies not just in its ability to identify differences and similarities, but in its capacity to generate insights that can inform better decisions and drive meaningful improvements across all areas of human endeavor.
FAQ: Comparative Analysis
What are the methods of comparative analysis?
The methods of comparative analysis involve evaluating and contrasting two or more entities to identify similarities and differences. Key methods include:
- Qualitative Comparative Analysis (QCA): Involves comparing case studies or qualitative data to identify patterns and causal relationships.
- Quantitative Comparative Analysis: Utilizes statistical methods to compare numerical data across different groups or time periods.
- Benchmarking: Comparing a company’s performance metrics against industry standards or competitors.
- SWOT Analysis: Comparing strengths, weaknesses, opportunities, and threats between different entities.
- Cross-Case Analysis: Used in research to compare multiple case studies to find common themes or differences.
What is an example of a comparative analysis?
An example of comparative analysis is comparing the financial performance of two companies in the same industry. By analyzing metrics such as revenue growth, profit margins, and return on investment, an analyst can determine which company is performing better and why. For instance, comparing the annual reports of Apple and Samsung to assess which company is more profitable and what factors contribute to their success.
What are comparative statistical analysis methods?
Comparative statistical analysis methods are used to compare datasets and determine if there are significant differences or relationships between them. Common methods include:
- T-tests: Used to compare the means of two groups and assess if the difference is statistically significant.
- ANOVA (Analysis of Variance): Compares the means of three or more groups to understand if at least one group differs significantly.
- Chi-square tests: Used to compare categorical data to see if there is an association between different variables.
- Regression analysis: Evaluates the relationship between dependent and independent variables across different groups.
- Correlation analysis: Measures the strength and direction of the relationship between two variables.
What are the three types of comparative analysis?
The three main types of comparative analysis are:
- Cross-Sectional Analysis: Compares data from different entities at a single point in time. For example, comparing the market share of different companies in a specific year.
- Longitudinal Analysis: Compares data over different time periods to identify trends, patterns, or changes. For instance, comparing a company’s revenue growth over the past five years.
- Cross-Cultural Analysis: Compares practices, beliefs, or behaviors across different cultures or societies. This type is often used in social sciences and international business studies.
What are the 4 principles of comparative analysis?
The four principles of comparative analysis that guide effective comparisons are:
- Relevance: Ensure that the entities being compared are relevant to the research question or objective.
- Consistency: Apply consistent criteria, methods, and standards when comparing different entities to ensure fairness and accuracy.
- Specificity: Focus on specific attributes or characteristics that are directly comparable across the entities.
- Contextualization: Understand and account for the context in which the entities operate, as this can impact the outcomes of the analysis.
What are the three methods of the comparative method?
The three methods of the comparative method often used in social sciences and research are:
- Most Similar Systems Design (MSSD): Compares cases that are similar in most aspects but differ in the outcome of interest. This method seeks to identify the factors that explain the differences in outcomes.
- Most Different Systems Design (MDSD): Compares cases that are different in most aspects but share the same outcome. This method aims to find the common factors that lead to the same result despite different conditions.
- Qualitative Comparative Analysis (QCA): A method that combines qualitative and quantitative approaches to compare multiple cases systematically, identifying patterns and causal relationships based on set theory.