Transcripts Model US
Transcripts Model US is a unique sentiment signal based on US earnings call transcripts. The model is generated using our tailor-made NLP engine which is trained recursively using a corpus of over 200,000 transcripts going back to 1999. We utilize the NLP engine to produce both Bag-of-words and word-embedding features, on which we train a machine learning model to predict future stock returns.
Using this signal, a portfolio that goes long the highest-ranking decile of liquid US stocks and short the bottom decile generates economically significant returns with low turnover. The signal has non-trivial factor and industry exposures. However, we show that after neutralizing both factor and industry tilts, the return predictive power becomes stronger and more consistent. The factor- and industry-neutralized signal generates an 13.7% annual return and a 2.57 Sharpe ratio over the entire sample (2006 – 2024) and was particularly effective in recent years.

The Transcripts Model is cross-sectionally robust; higher-ranked stocks in our simple 1-to-100 percentile scores correspond to higher future annualized returns, both before and after controlling for the risk factors in the ExtractAlpha Risk Model, with nearly monotonic returns for both raw and residualized returns across decile buckets:
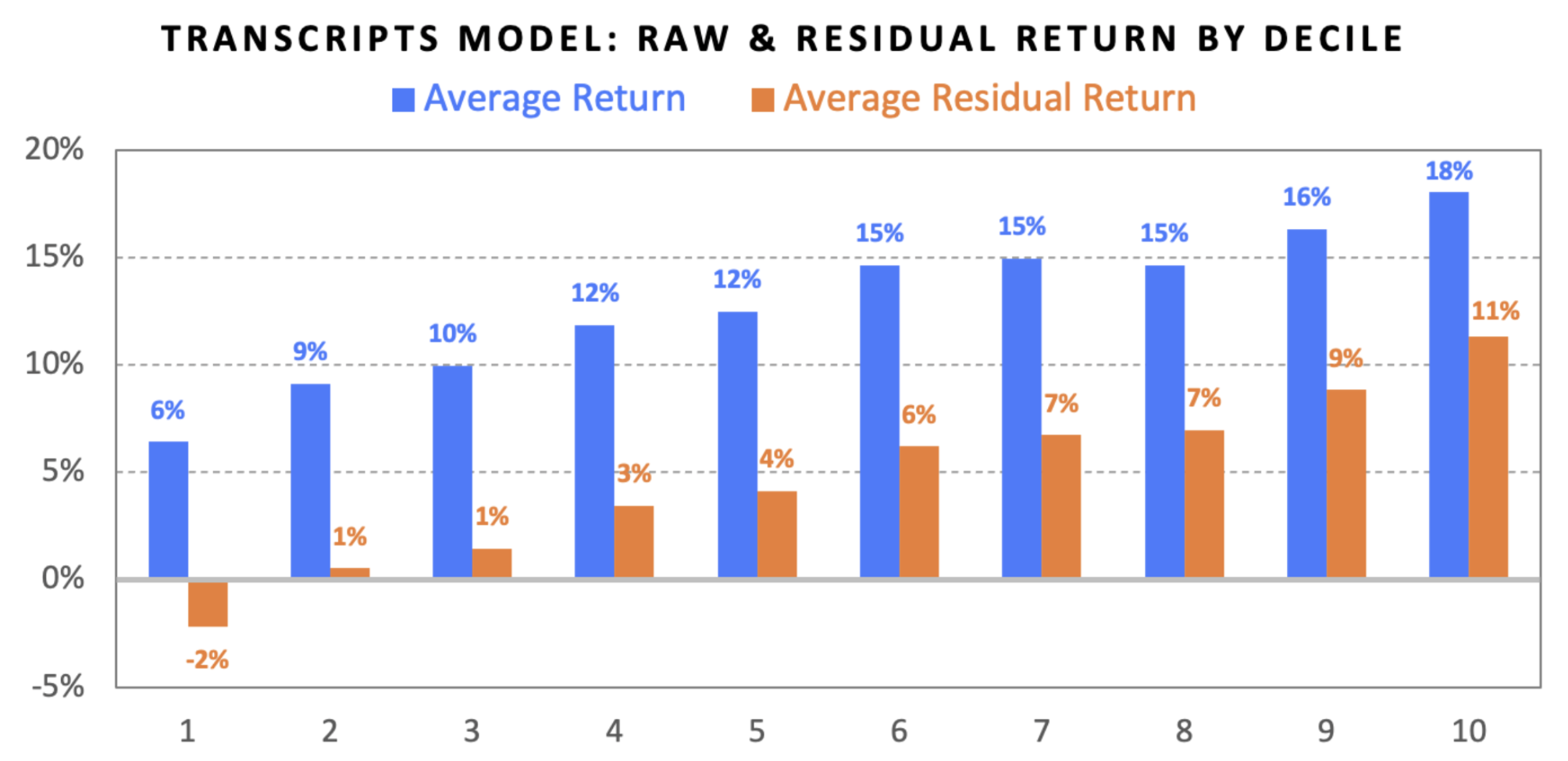