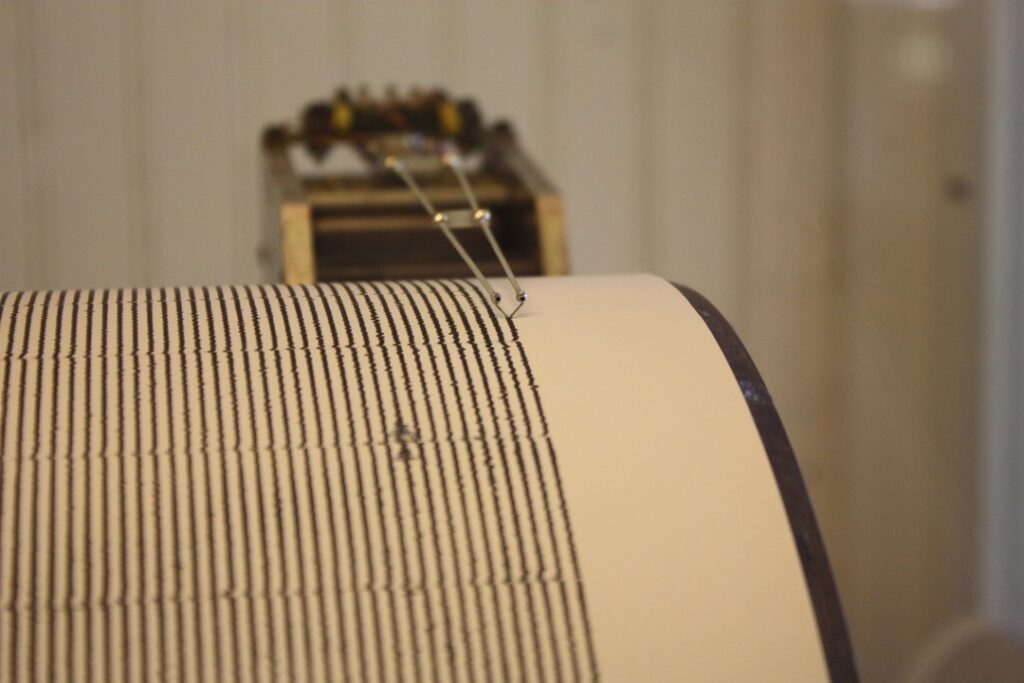
August 7, 2017. 10 years ago today there was a wakeup call in systematic investing when many quants across the Street suffered their worst losses – before or since – over a three day period that has been called the “Quant Quake.” The event wasn’t widely reported outside of the quant world, but it was a worldview-changing week for those of us who traded through it. Most quant investors today, it seems, either didn’t hear the wakeup call, or have forgotten. This article addresses what’s changed in the last ten years, what hasn’t, what we learned and what we didn’t, and eight ideas on how to change your research process to insulate yourself from the next Quake.
In summary:
- The Quake, which caused massive losses in quant funds in 2007 as well as some fund closures, was driven by crowded trades and similar alphas across many funds
- There are more quants trading more capital today than ten years ago, but most of them haven’t significantly changed their alphas or data sources and have not widely adopted alternative data, perhaps due to complacency or herding behavior
- So there’s more risk of another Quake than there was 10 years ago. In 2017 we’re seeing evidence of crowdedness and poor performance in standard strategies, with alternative data sets exhibiting far stronger performance
- Quants need to build systematic processes for evaluating new data sources, and should view alternative data as a prime directive
The Quake
After poor but not hugely unusual performance in July ’07, many quantitative strategies experienced dramatic losses – 12 standard deviation events or more by some accounts – over the three consecutive days of August 7, 8, and 9. In the normally highly risk controlled world of market neutral quant investing, such a string of returns was unheard of. Typically-secretive quants even reached out to their competitors to get a handle on what was going on, though no clear answers were immediately forthcoming.
Many quants believed that the dislocations must be temporary since they were deviations from what the models considered fair value. During the chaos, however, each manager had to decide whether to cut capital to stem the bleeding – thereby locking in losses – or to hang on and risk having to close shop if the expected snap back didn’t arrive on time. And the decision was sometimes not in their hands, in cases where they didn’t have access to steady sources of capital. Hedge funds with monthly liquidity couldn’t be compelled by their investors to liquidate, but managers of SMAs and proprietary trading desks didn’t necessarily have that luxury.
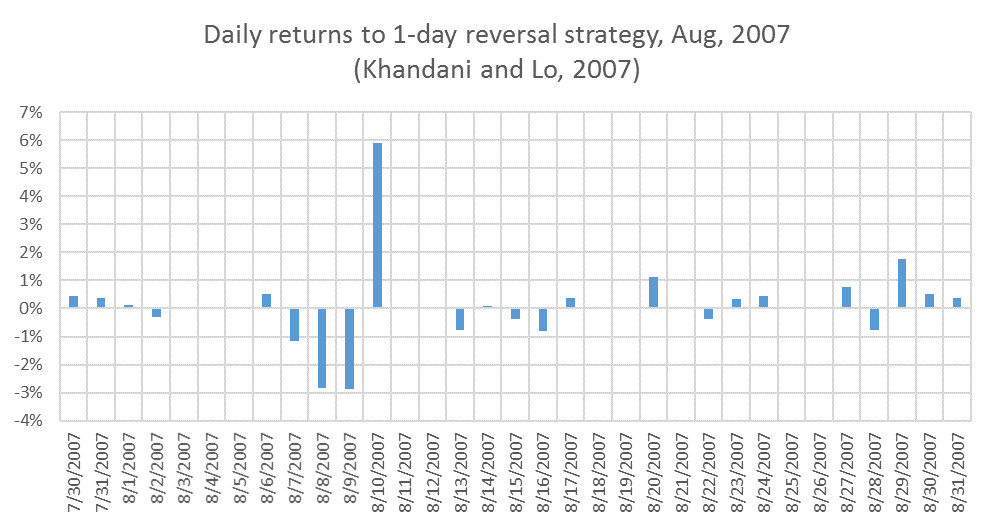
On August 10th, the strategies rebounded strongly, per the chart above from Khandani and Lo’s postmortem Quant Quake paper. By the end of the week, those quants who had held on to their positions were nearly back where they started; their monthly return streams wouldn’t even register a blip! Unfortunately, many hadn’t, or couldn’t, hold on; they cut capital or reduced leverage – in some cases, like GSAM, to this day. Some large funds shut down soon afterwards.
What happened???
Gradually a sort of consensus emerged about what had happened. Most likely, a multi-strategy fund which traded both classic quant signals and some less liquid strategies suffered some large losses in those less liquid books; and they liquidated their quant books quickly to cover the margin calls. The positions they liquidated turned out to be very similar to the positions held by many other quant-driven portfolios across the world; and the liquidation put downward pressure on those particular stocks, thereby negatively affecting other managers, some of whom in turn liquidated, causing a domino effect. Meanwhile, the broader investment world didn’t notice; these strategies were mostly market neutral and there were no large directional moves in the market at the time.
With hindsight, we can look back at some factors which we knew to have been crowded and some others which were not, and see the difference in performance during the Quake quite clearly. In the chart below, we look at three crowded factors: earnings yield; 12-month price momentum; and 5-day price reversal. Most of the data sets we now use to reduce the crowdedness of our portfolios weren’t around in 2007, but for a few of these less-crowded alphas we can go back that far in a backtest. Here, we use components of some ExtractAlpha models, namely: the Tactical Model (TM1)’s Seasonality component, which measures the historical tendency of a stock to perform well at that time of year; the Cross-Asset Model (CAM1)’s Volume component, which compares Put to Call volume and option to stock volume; and CAM1’s Skew component, which measures the implied volatility of out of the money puts. The academic research documenting these anomalies was mostly published between 2008 and 2012, and the ideas weren’t very widely known at the time; arguably, these anomalies are still relatively uncrowded compared to their “Smart Beta” counterparts.
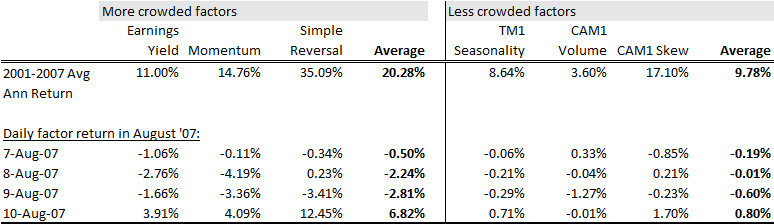
The table above shows the average annualized return of dollar neutral, equally weighted portfolios of liquid U.S. equities built from these single factors and rebalanced daily. For the seven-year period up to and through the Quant Quake, the less crowded factors didn’t perform spectacularly, on average, whereas the crowded factors did quite well; their average annualized return for the period was around 10% before costs, about half that of the crowded factors. But their drawdowns during the Quake were minimal, compared to those of the crowded factors. Therefore, we can view some of these factors as diversifiers or hedges against crowding. And to the extent that one does want to unwind positions, there should be more liquidity in a less-crowded portfolio.
It turned out, we were all trading the same stuff!
The inferior performance of the factors which we now know to have been crowded was a shocking revelation to some managers at the time who viewed their methodology as unique or at least uncommon. It turned out, we were all trading the same stuff! Most equity market neutral quants traded pretty much the same universe, controlling risk using pretty much the same risk models… and pretty much betting on the same alphas built on the same data sources! In many ways, the seed of the idea which became ExtractAlpha – that investors need to diversify their factor bets beyond these well-known ones – were planted in 2007. At the time one would have assumed that other quants would have had the same thought, and that the Quant Quake was a call to arms – but as we’ve learned more recently, the arms don’t seem to have been taken up.
But it won’t happen again… will it?
Quant returns were generally good in the ensuing years, but many groups took years to rehabilitate their reputations and AUMs. By early 2016, the Quant Quake seemed distant enough, and returns had been good enough for long enough that complacency had set in. Times were good – until they weren’t, as many quant strategies have fared poorly in the last 18 months. At least one sizable quant fund has closed, and several well known multi-manager firms have shut their quant books. Meanwhile, many alternative alphas have done well. In our view, this was somewhat inevitable; since 2013 we’ve been saying that times eventually wouldn’t be good due to recent crowding in common quant factors, in part due to the proliferation of quant funds, their decent performance relative to discretionary managers, and the rise of smart beta products; and there’s a clear way to protect yourself: diversify your alphas!
With so much data available today, there’s no excuse for letting your portfolio be dominated by crowded factors.
With so much data available today – most of which was unavailable in 2007 – there’s no longer any excuse for letting your portfolio be dominated by classic, crowded factors. Well, maybe some excuses. Figuring out which data sets are useful is hard. Turning them into alphas is hard. But we’ve had ten years to think about it now. These are the problems ExtractAlpha helps its clients solve, by parsing through dozens of unique data sets and turning them into actionable alphas.
You’d think quants would actively embrace new alpha sources, and would have started doing so in earnest around August 15th, 2007. Strangely, they barely seem to have done so at all. Most quant managers still rely on the same factors they always have, though they may trade them with more attention to risk, crowding, and liquidity. Alternative data hasn’t crossed the chasm.
Perhaps the many holdouts are simply hoping that value, momentum, and mean reversion aren’t really crowded, or that their take on these factors really is sufficiently differentiated – which it may be, but it seems a strange thing to rely on in the absence of better information. It’s also true that there are a lot more quants and quant funds around now than there were then, across more geographies and styles – and so the institutional memory has faded a lot. Those of us who were trading in those days are veterans (and we don’t call ourselves “data scientists” either!)
It’s also possible that a behavioral explanation is at work: herding. Just like allocators who pile money into the largest funds despite those funds’ underperformance relative to emerging funds – because nobody can fault them for a decision everyone else has also already made – or like research analysts who only move their forecasts with the crowd to avoid a bold, but potentially wrong, call – perhaps quants prefer to be wrong at the same time as everyone else. Hey, everyone else lost money too, so am I so bad? This may seem to some managers to be a better outcome than adopting a strategy which is more innovative than using classic quant factors but which has a shorter track record and is potentially harder to explain to an allocator.
Another quant quake is actually more likely now than it was ten years ago.
Whatever the rationale, it seems clear that another quant quake is actually more likely now than it was ten years ago. The particular mechanism might be different, but a crowdedness-driven liquidation event seems very possible in these crowded markets.
So, what should be done?
We do see that many funds have gotten better at reaching out to data providers and working through the evaluation process in terms of vendor management. But most have not become particularly efficient at evaluating the data sets in the sense of finding alpha in them.
In our view, any quant manager’s incremental research resources should be applied directly towards acquiring orthogonal signals (and, relatedly, to controlling crowdedness risk) rather than towards refining already highly correlated ones in order to make them possibly slightly less correlated. Here are eight ideas on how to do so effectively:
- The focus should be on allocating research resources specifically to new data sets, setting a clear time horizon for evaluating each (say, 4-6 weeks), and making a definitive call about the presence or absence of added value from a data set. This requires maintaining a pipeline of new data sets and sticking to a schedule and a process.
- Quants should build a turnkey backtesting environment which can efficiently evaluate new alphas and determine their potential added value to the existing process. There will always be creativity involved in testing data sets, but the more mundane data processing, evaluation, and reporting aspects should be automated to expedite the process in (1)
- An experienced quant should be responsible for evaluating new data sets – someone who has seen a lot of alpha factors before and can think about how the current one might be similar or different. New data sets shouldn’t be a side project, but rather a core competency of any systematic fund.
- Quants should pay attention to innovative data suppliers rather than what’s available from the big players (admittedly, we’re biased on this one!)
- Priority should be given to data sets which are relatively easy to test, in order to expedite one’s exposure to alternative alpha. More complex, raw, or unstructured data sets can indeed get you to more diversification and more unique implementations, but at the cost of sitting on your existing factors for longer – so it’s best to start with some low hanging fruit if you’re new to alternative data
- Quants need to gain comfort with limited history that we often see with alternative data sets. We recognize that with many new data sets one is “making a call” subject to limited historical data. We can’t judge these data sets by the same criteria of 20-year backtests as we can with more traditional factors, both because the older data simply isn’t there and because the world 20 years ago has little bearing on the crowded quant space of today. But the alternative sounds far more risky.
- In sample and out of sample methodologies might have to change to account for the shorter history and evolving quant landscape.
- Many of the new alphas we find are relatively short horizon compared to their crowded peers; the alpha horizons are often in the 1 day to 2 month range. For large-AUM asset managers who can’t be too nimble, using these faster new alphas in unconventional ways such as trade timing or separate faster-trading books can allow them to move the needle with these data sets. We’ve seen a convergence to the mid-horizon as quants who run lower-Sharpe books look to juice their returns and higher-frequency quants look for capacity, making the need for differentiated mid-horizon alphas even greater.
I haven’t addressed risk and liquidity here, which are two other key considerations when implementing a strategy on new or old data. But for any forward-thinking quant, sourcing unique alpha should be the primary goal, and implementing these steps should help to get them there. Let’s not wait for another Quake before we learn from the lessons of ten years ago!
Originally posted on LinkedIn at https://www.linkedin.com/pulse/quant-quake-10-years-vinesh-jha