Much of our research at ExtractAlpha focuses on earnings events, and several of our alternative data products give investors a variety of views into a company’s earnings and revenues, for example through the lens of digital footprint via our Digital Revenue Signal; sell side estimates via our TrueBeats; or crowd intelligence via our Estimize platform. Today we are excited to announce our latest way for investors to analyze corporate earnings by way of textual data from earnings call transcripts.
Over the last two years we’ve been researching applications of Natural Language Processing (NLP) to transcripts, and we believe we’ve built a model which is both robust (its predictive power increases substantially when controlling for risk exposures) and scalable (its turnover is substantially lower than other commercial sentiment signals based on NLP.
The Transcripts Model is generated using our tailored NLP engine which is trained recursively using a corpus of over 200,000 transcripts from FactSet going back to 1999. We utilize the NLP engine to produce both traditional dictionary-based features and features which use more advanced machine learning approaches, which we further link to future stock returns through an additional machine learning process. The resulting model passes intuition-based sniff tests, with “positive-sounding” transcript language correlating with higher scores and “negative-sounding” language correlating with lower scores.
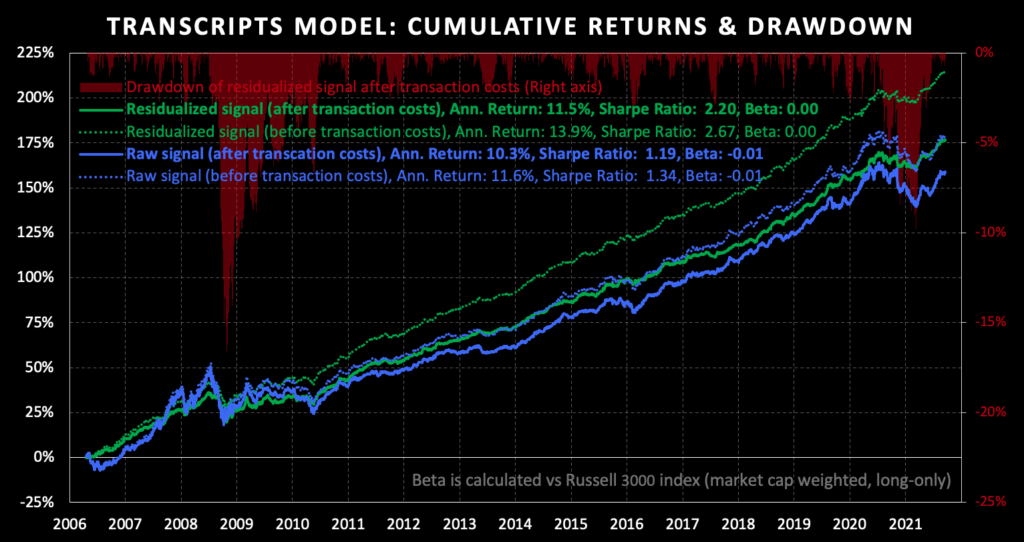
A portfolio that goes long the highest-ranking decile of liquid U.S. stocks and short the bottom decile according to the Transcripts Model generates economically significant returns with low turnover. After neutralizing both factor and sector tilts, the portfolio generates a 13.9% annual return and a 2.67 Sharpe ratio before transaction costs over the entire sample (2006 – 2021) and has been particularly effective in recent years. Furthermore, performance after transaction costs are similar to before transaction costs since daily turnover is low at only 1.7%. The chart at the top of this page shows these cumulative returns.
There is cross-sectional robustness too; higher-ranked stocks in our simple 1-to-100 percentile scores correspond to higher future annualized returns, both before and after controlling for the risk factors in the ExtractAlpha Risk Model, with nearly monotonic returns for both raw and residualized returns across decile buckets:
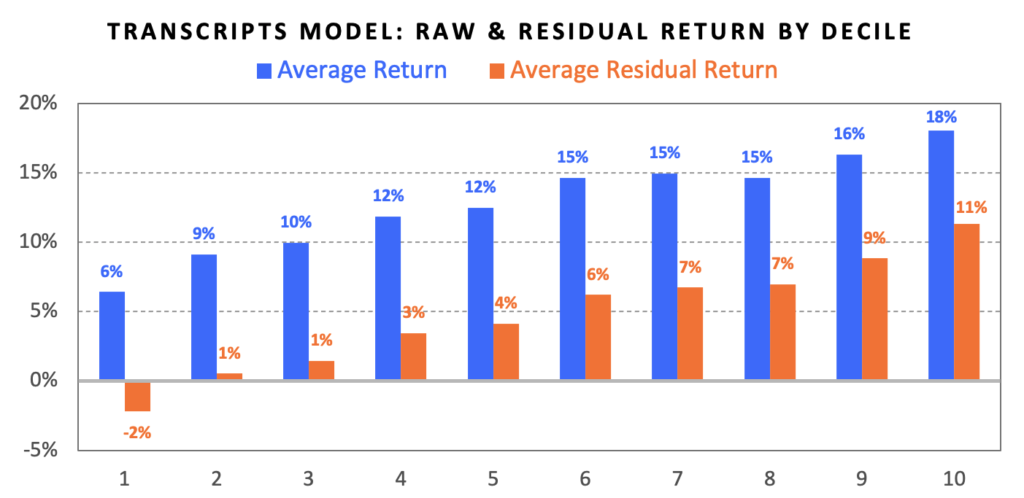
As such we believe the Transcripts Model will be additive to existing multi-factor quant processes on timescales of days to months.
As with all of our data products, we offer free white papers and historical trials of the data to qualified institutional investors.