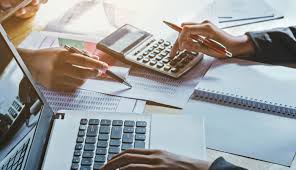
Reinforcement Learning (RL) is a branch of machine learning where an agent learns to make decisions by interacting with an environment.
In the context of finance, RL has emerged as a powerful tool for tackling complex, dynamic problems that traditional methods struggle to solve effectively.
The relevance of reinforcement learning to the finance industry stems from its ability to adapt to changing market conditions, handle high-dimensional data, and make sequential decisions under uncertainty.
These characteristics make RL particularly suited to financial applications such as trading, portfolio management, and risk assessment.
Fundamentals of Reinforcement Learning
Key components
Reinforcement learning systems consist of several key components:
- Agent: The decision-making entity that learns from the environment
- Environment: The financial market or system in which the agent operates
- States: The current situation or condition of the environment
- Actions: Decisions the agent can make (e.g., buy, sell, hold)
- Rewards: Feedback signals indicating the desirability of actions
Basic algorithms
Several foundational algorithms form the basis of RL in finance:
- Q-learning: A model-free algorithm that learns the value of actions in different states
- SARSA (State-Action-Reward-State-Action): An on-policy learning algorithm
- Policy Gradients: Methods that directly optimize the policy without using a value function
Deep reinforcement learning
Deep RL combines reinforcement learning with deep neural networks, allowing for more complex representations and the ability to handle high-dimensional state spaces common in financial data.
Applications in Finance
Algorithmic trading
RL can be used to develop adaptive trading strategies that evolve with market conditions. Agents can learn to identify profitable trading opportunities and execute orders optimally.
Portfolio management
Reinforcement learning algorithms can dynamically adjust portfolio allocations based on changing market conditions, risk preferences, and investment goals.
Risk management
RL models can assess and manage various types of financial risks, including market risk, credit risk, and operational risk, by learning from historical data and simulations.
Market making
In market making, RL agents can learn to set bid-ask spreads and manage inventory to maximize profits while providing liquidity to the market.
Fraud detection
RL can be applied to detect fraudulent activities by learning patterns of normal and suspicious behaviors in financial transactions.
Advantages of Reinforcement Learning in Finance
Adaptability to changing market conditions
RL models can continuously learn and adapt to new market dynamics, making them more robust than static models.
Ability to handle complex, multi-dimensional problems
Financial markets involve numerous interrelated variables. RL can effectively navigate these high-dimensional spaces.
Potential for automated decision-making
Once trained, RL agents can make rapid, data-driven decisions without human intervention, potentially reducing emotional biases.
Continuous learning and improvement
RL models can be designed to learn from new data in real-time, continuously improving their performance.
Challenges and Limitations
Data quality and availability
Financial data can be noisy, incomplete, or subject to survivorship bias, presenting challenges for RL model training.
Interpretability and explainability
The complexity of RL models, especially deep RL, can make it difficult to explain their decision-making processes, which is crucial in regulated financial environments.
Regulatory compliance
The use of AI in financial decision-making is subject to increasing regulatory scrutiny, requiring careful consideration of compliance issues.
Overfitting and generalization issues
RL models may perform well in training environments but fail to generalize to real-world market conditions.
Case Studies
Successful implementations in trading firms
Several hedge funds and proprietary trading firms have reported success using RL for algorithmic trading, although specific details are often kept confidential.
Research breakthroughs in financial applications
Academic research has demonstrated the potential of RL in areas such as optimal execution of large orders and dynamic asset allocation.
Comparison with Traditional Methods
Reinforcement learning vs. supervised learning
Unlike supervised learning, RL doesn’t require labeled data and can learn from trial and error, making it more suitable for dynamic financial environments.
Advantages over rule-based systems
RL can discover complex strategies that may not be apparent to human experts, potentially outperforming traditional rule-based systems.
Ethical Considerations
Potential for market manipulation
The autonomous nature of RL agents raises concerns about potential market manipulation or unintended disruptive behaviors.
Fairness and bias in financial decision-making
RL models may inadvertently learn and perpetuate biases present in historical financial data.
Impact on human jobs in finance
The automation potential of RL in finance raises questions about its impact on employment in the financial sector.
Tools and Frameworks
Popular libraries
Several open-source libraries support RL development in finance:
- TensorFlow and Keras-RL
- PyTorch and Stable Baselines3
- OpenAI Gym for creating RL environments
Specialized platforms for financial reinforcement learning
Some companies offer specialized platforms that combine financial data feeds with RL capabilities tailored for trading and investment applications.
Future Trends
Integration with other AI technologies
The combination of RL with natural language processing and computer vision could lead to more comprehensive financial decision-making systems.
Quantum reinforcement learning in finance
Quantum computing may eventually enhance RL algorithms, potentially revolutionizing areas like portfolio optimization and risk management.
Federated learning for collaborative model development
Federated learning techniques could allow financial institutions to collaboratively train RL models without sharing sensitive data.
Best Practices for Implementation
Data preparation and feature engineering
Careful preprocessing and feature selection are crucial for the success of RL models in finance.
Model selection and hyperparameter tuning
Choosing the right RL algorithm and optimizing its parameters is essential for achieving good performance.
Backtesting and validation strategies
Rigorous backtesting and out-of-sample validation are necessary to ensure RL models are robust and generalize well to unseen market conditions.
Extract Alpha and Reinforcement Learning
Extract Alpha datasets and signals are used by hedge funds and asset management firms managing more than $1.5 trillion in assets in the U.S., EMEA, and the Asia Pacific. We work with quants, data specialists, and asset managers across the financial services industry.
In the context of reinforcement learning in finance, Extract Alpha’s expertise in data analysis and signal generation can be particularly valuable. The company’s advanced methodologies can be applied to:
- Develop high-quality state representations for RL agents using alternative data sources
- Design reward functions that align with real-world financial objectives
- Create realistic simulation environments for training and testing RL models
- Generate features and signals that can enhance the performance of RL-based trading strategies
- Provide benchmarks and performance metrics for evaluating RL models against traditional approaches
As reinforcement learning continues to gain traction in the financial industry, the sophisticated data analysis techniques employed by firms like Extract Alpha are likely to play an increasingly important role in developing and refining RL applications in finance.
Conclusion
Reinforcement learning holds transformative potential for the finance industry, offering new ways to approach complex problems in trading, investment, and risk management. Its ability to adapt to changing market conditions and make decisions in high-dimensional, uncertain environments makes it a powerful tool for financial professionals.
However, the implementation of RL in finance is not without challenges. Issues of data quality, model interpretability, and regulatory compliance must be carefully addressed. Moreover, ethical considerations regarding market fairness and the societal impact of AI in finance need to be at the forefront of development efforts.
As the field progresses, we can expect to see more sophisticated RL applications in finance, potentially reshaping how financial decisions are made. The key to success will lie in balancing the innovative potential of RL with robust risk management practices and ethical considerations.
The future of reinforcement learning in finance is promising, but it requires a thoughtful approach that combines technical expertise with a deep understanding of financial markets and their societal impact. As financial institutions continue to explore and implement RL solutions, they must strive to harness its power responsibly, ensuring that the benefits of this technology are realized while mitigating potential risks.
FAQ: Machine Learning and Reinforcement Learning in Finance
How to use ML in finance?
Machine Learning (ML) is used in finance to enhance decision-making, improve efficiency, and manage risk. Common applications include:
- Algorithmic trading: ML algorithms analyze market data in real-time to execute trades automatically based on predictive models.
- Credit scoring: ML models assess the creditworthiness of individuals or businesses by analyzing historical data and patterns.
- Fraud detection: ML systems identify suspicious transactions by detecting anomalies and unusual patterns in financial data.
- Portfolio management: ML helps in optimizing asset allocation by predicting market trends and assessing risk.
- Customer service: Chatbots and AI-driven assistants use ML to provide personalized financial advice and support.
How is reinforcement learning used in trading?
Reinforcement learning (RL) is used in trading by enabling algorithms to learn optimal trading strategies through trial and error. The RL agent interacts with the trading environment, making decisions such as buying or selling assets, and receives feedback in the form of rewards (e.g., profit) or penalties (e.g., loss). Over time, the RL model improves its strategy to maximize returns by learning from its past actions.
What is an example of supervised learning in finance?
An example of supervised learning in finance is credit scoring. In this case, a supervised learning algorithm is trained on historical data, where the input features might include an individual’s income, credit history, and employment status, and the output is the credit score or loan approval status. The algorithm learns the relationship between the inputs and outputs and can then predict the creditworthiness of new applicants.
How is reinforcement learning used in business?
Reinforcement learning is used in business to optimize decision-making in dynamic environments. Examples include:
- Supply chain management: RL models can optimize inventory levels, reduce costs, and improve logistics by learning the best strategies over time.
- Marketing strategies: RL helps in determining the best marketing actions by learning from customer interactions and responses to various campaigns.
- Personalized recommendations: RL systems can tailor product or service recommendations to individual customers by learning their preferences and behavior patterns.
How is reinforcement learning used in finance?
In finance, reinforcement learning is applied to develop strategies that adapt to changing market conditions. Applications include:
- Algorithmic trading: RL models learn to make buy, sell, or hold decisions in real-time to maximize portfolio returns.
- Risk management: RL can help in optimizing risk-adjusted returns by learning how to allocate assets under different market scenarios.
- Portfolio optimization: RL algorithms learn to balance the risk and return of a portfolio by continuously adapting to market changes and investor preferences.
Which type of problems can be solved by reinforcement learning?
Reinforcement learning can solve problems that involve decision-making in complex, dynamic environments where the goal is to maximize a long-term reward. Examples include:
- Trading strategies: Developing algorithms that adapt to changing market conditions.
- Robotics: Teaching robots to perform tasks like walking or assembling parts by learning from trial and error.
- Game playing: RL has been used to develop AI that can beat human champions in games like chess, Go, and poker.
- Resource management: Optimizing the allocation of limited resources in areas like energy distribution, telecommunications, or healthcare.
- Autonomous vehicles: Enabling self-driving cars to learn how to navigate safely in various traffic conditions.