Introduction
In the ever-evolving world of finance, hedge funds employing quantitative strategies have become trailblazers, reshaping investment landscapes with mathematical precision. This article delves into the intricacies of quantitative strategies in hedge funds, unveiling the methodologies that fuel their success.
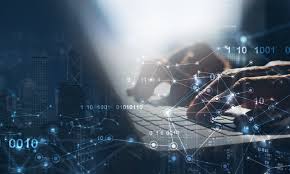
Understanding Quantitative Strategies
A Foundation Before delving into the specifics, let’s establish a foundation by understanding what quantitative strategies entail. This section provides an overview of the principles that underpin these strategies, emphasizing the role of mathematical models, algorithms, and data analysis in the decision-making process.
1. Momentum Trading
Riding the Waves of Success Momentum trading is a pivotal component of many quantitative hedge fund strategies. This section explores how funds leverage statistical analysis and mathematical models to identify and capitalize on existing market trends, riding the waves of momentum for profitable outcomes.
2. Statistical Arbitrage
Capitalizing on Market Inefficiencies Statistical arbitrage involves exploiting pricing discrepancies between related financial instruments. This section delves into how quantitative hedge funds utilize sophisticated models and algorithms to identify mispricings, execute trades, and capitalize on market inefficiencies.
3. Algorithmic Trading
Precision and Speed in Execution Algorithmic trading encompasses various quantitative strategies. This section explores how hedge funds leverage algorithms to execute trades with precision and speed, gaining a competitive edge in today’s fast-paced financial markets.
4. Machine Learning
Unleashing the Power of Data Machine learning has revolutionized quantitative hedge fund strategies, allowing funds to analyze vast datasets and make data-driven predictions. This section explores how machine learning algorithms enhance decision-making and contribute to the success of these funds.
Estimize
Before we explore further, it’s important to highlight Estimize, a groundbreaking platform that leverages the collective wisdom of over 100,000 contributors worldwide. Estimize provides the world’s most accurate earnings and macroeconomic estimates, welcoming a diverse range of contributors, including buy-side, independent, and sell-side professionals, as well as amateur analysts, independent investors, and academics. Register as a contributor or get access to the Estimize data set at estimize.com.
Challenges in Quantitative Hedge Fund Strategies
Navigating Complexities While quantitative strategies offer powerful tools, they are not without challenges. This section explores considerations such as model risk, data quality, and the ongoing need for adaptation to market dynamics.
The Global Impact
Shaping Markets Across Borders Quantitative hedge fund strategies operate on a global scale, influencing markets beyond their geographical origins. This section examines how these strategies contribute to the interconnected nature of international financial markets.
The Future of Quantitative Hedge Fund Strategies
Trends and Innovations As technology evolves, so too do quantitative hedge fund strategies. This section explores emerging trends, including the integration of artificial intelligence, machine learning advancements, and the growing importance of environmental, social, and governance (ESG) factors in quantitative models.
Conclusion
Quantitative hedge fund strategies are not merely tools; they are the vanguard of financial evolution. As we navigate the complexities of markets, understanding and appreciating the nuances of these strategies becomes paramount for investors aiming to stay ahead.
Frequently Asked Questions
1. What are the primary advantages of using quantitative strategies in hedge funds?
Quantitative strategies in hedge funds offer several advantages, including the ability to process vast amounts of data, remove emotional bias from trading decisions, and identify subtle patterns in market behavior that may be invisible to human traders. These strategies also enable faster decision-making and can improve the consistency of investment returns.
2. How do quantitative hedge funds address the risk of model overfitting?
Quantitative hedge funds mitigate the risk of model overfitting by employing robust backtesting procedures, using out-of-sample data testing, and continuously monitoring the performance of their models against real-world outcomes. Additionally, they often use ensemble methods that combine multiple models to reduce reliance on any single predictive framework.
3. Can individual investors apply quantitative strategies, or are they only viable for hedge funds?
Individual investors can indeed apply some quantitative strategies, especially those that involve basic algorithmic trading and statistical analysis. However, the more complex strategies, particularly those requiring significant computational power and data access, might be more challenging to implement without the resources of a hedge fund.
4. What role does machine learning play in modern quantitative hedge funds?
Machine learning plays a crucial role in modern quantitative hedge funds by enhancing their ability to forecast market movements and identify profitable trading opportunities. Machine learning algorithms can adapt to new data and learn from previous trades to improve their predictions, making them invaluable in dynamic market environments.
5. Are there ethical concerns associated with quantitative trading, and how are they addressed?
Ethical concerns in quantitative trading include the potential for market manipulation and the exacerbation of market volatility. Regulatory bodies and hedge funds address these concerns by implementing strict compliance measures and transparency requirements. Additionally, many funds now incorporate ethical considerations into their algorithms to ensure their trading activities contribute positively to market integrity.